Understanding Video Labeling: Techniques and Applications
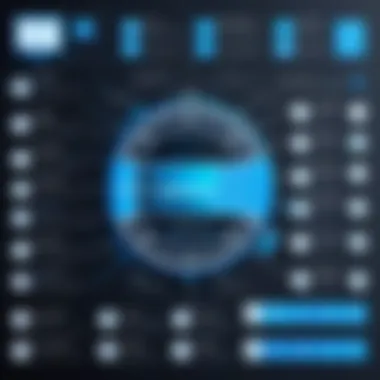
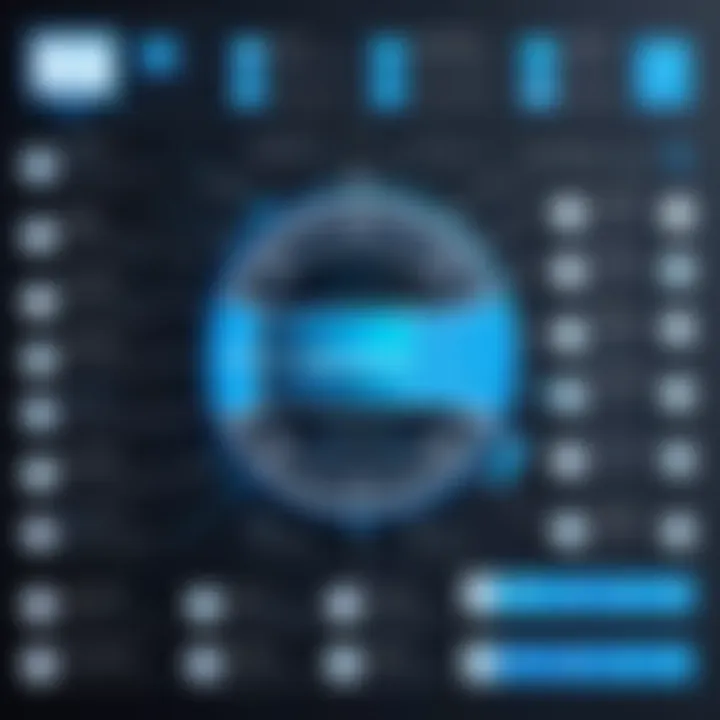
Intro
Video labeling is a foundational technique in the realm of machine learning and artificial intelligence. As more industries look to harness the power of video data, the necessity for effective labeling methodologies has become increasingly apparent. Video labeling allows for the annotation of key events, objects, and actions within videos, serving as a significant resource for training algorithms. In this article, we delve into the techniques and challenges associated with video labeling while exploring its applications across various sectors.
Understanding video labeling is essential for tech-savvy individuals and business professionals who aim to implement data-driven insights within their organizations. It sheds light on how to derive value from large video datasets and enhances decision-making processes within technology-based environments.
Software Needs Assessment
A critical step in video labeling is assessing the software requirements based on intended use cases. This ensures that the video labeling tools selected align with both user needs and technical specifications.
Identifying User Requirements
User requirements must be clearly outlined to find the right tools for video labeling. These requirements can vary significantly based on the objectives of the organization. Common factors to consider include:
- Type of video data: Analyzing live streams versus recorded footage may necessitate different approaches.
- Integration capabilities: The labeling software should integrate seamlessly with existing systems for better workflow.
- Scalability: Ability to handle increasing volumes of data over time is crucial.
- User-friendliness: Software must accommodate various skill levels among staff.
Evaluating Current Software Solutions
With requirements in hand, evaluating current software solutions becomes a systematic process. It is important to analyze several key software attributes:
- Feature set: Does the software offer required tools for specific labeling tasks?
- Support and community: Access to user support and an active community can enhance the user experience.
- Cost-effectiveness: Assess if the softwareโs pricing aligns with the budget and anticipated ROI.
For instance, solutions like Labelbox and VGG Image Annotator serve different functionalities and user experiences, making comparisons necessary.
Data-Driven Insights
In the age of information, the ability to leverage data-driven insights through video labeling is profound. Insights derived from video data can inform strategic decisions across sectors.
Market Trends Overview
The landscape of video labeling continues to evolve. As organizations seek to exploit video data, trends such as automated labeling are gaining traction. Companies are increasingly adopting AI-driven tools to assist humans in the labeling process, leading to faster decision-making capabilities.
Performance Metrics
Establishing performance metrics is essential in understanding the efficacy of video labeling processes. Key metrics to assess the impact include:
- Accuracy of labels: The precision in identifying and annotating elements within video.
- Time efficiency: Measuring the duration taken to complete labeling tasks.
- User satisfaction: Gauging how effectively teams can utilize the labeled datasets for their needs.
Understanding these elements forms the backbone of an effective video labeling strategy and can significantly influence organizational outcomes.
"Investing in proper software tools for video labeling is not just a choice, it is a necessity for organizations aiming for data-driven success."
Video labeling is not merely a technical hurdle; it is a gateway to unlocking the potential within video data, leading to smarter business decisions and advancements in technology.
Prelims to Video Labeling
Video labeling is a core process in the realm of video data annotation. This section elucidates how vital video labeling is, particularly in the context of machine learning and artificial intelligence. As technology continues to advance, the need for precise and accurate video labeling methods becomes ever more critical. The ability to train algorithms effectively hinges on the quality of labeled data, making video labeling not just a routine task, but a pivotal component of data-driven decision making.
A well-defined video labeling process enhances machine learning models' efficacy across various applications. This efficiency is achieved by providing the necessary contextual information that machines need to learn from the data. Without properly labeled videos, algorithms may fail to recognize patterns or make informed decisions, ultimately impacting their performance in practical applications.
Each technique in video labeling presents unique advantages and challenges, whether involving manual annotation, automated methods, or hybrid approaches. Being mindful of these techniques is essential for organizations that aim to leverage video data for insights and innovations.
Definition and Importance
Video labeling refers to the process of tagging or annotating segments of video data with relevant information. This could include labeling objects, actions, or events observed in the footage. The importance of video labeling cannot be overstated; it is the foundation upon which machine learning models operate. By transforming raw video feeds into structured data, labeling provides necessary insights that directly influence the capabilities of AI systems.
In practical terms, video labeling has a broad range of applications, including autonomous vehicles, healthcare diagnostics, and security surveillance. For example, an autonomous vehicle relies on labeled video data to identify pedestrians, obstacles, and navigational cues. Without accurate labels, the vehicleโs ability to operate safely and efficiently would be compromised. Thus, ensuring high-quality video labeling is critical for success in these fields.
Historical Context and Evolution
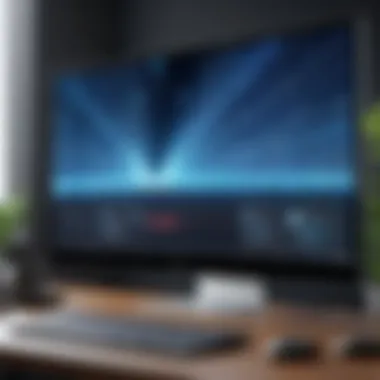
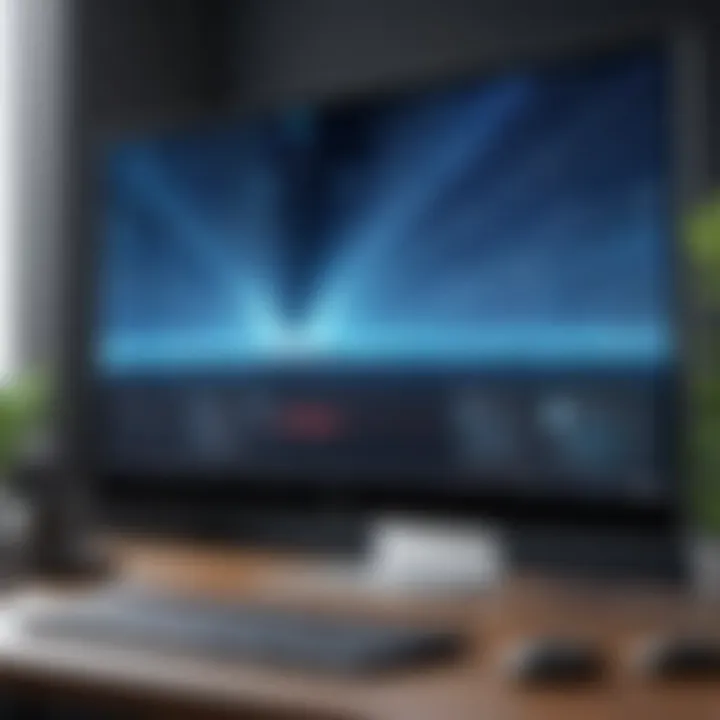
The concept of video labeling has evolved significantly over time. Initially, video annotation was performed manually. Early annotators would painstakingly review footage, frame by frame, to assign labels. This method was labor-intensive and often led to inconsistencies in labeling, which affected the quality of data for machine training.
With the advent of machine learning and computer vision algorithms, automated labeling techniques began to emerge. These methods utilize pre-trained models to identify and tag elements in video, significantly reducing time and human effort. However, while automation decreases the workload, it does not eliminate the need for human oversight, especially for complex or nuanced labeling tasks.
Today, hybrid approaches that combine both manual and automated techniques are gaining traction. They aim to optimize the efficiency and accuracy of video labeling by leveraging the strengths of both methods. Ensuring that technological advancements continue to shape the future of video labeling is crucial, as more sophisticated applications arise in diverse sectors.
Core Techniques in Video Labeling
Video labeling is critical for optimizing the performance of machine learning models. The core techniques used in this process play a vital role in ensuring that algorithms receive accurate and well-structured data for training. These techniques can be broken down into three primary categories: manual video annotation, automated video labeling methods, and hybrid approaches. Each of these methods has its unique strengths and vulnerabilities, which are essential for professionals to consider when choosing a strategy for their specific needs.
Manual Video Annotation
Manual video annotation is perhaps the most traditional technique in the field. It involves human annotators watching the video content and identifying specific elements or events. This technique is often favored for its accuracy, as human judgment can capture subtle nuances that machines may not yet comprehend fully. Annotators apply labels and tags based on defined criteria, enabling high levels of precision in the annotations. This method is particularly valuable in contexts where context and understanding are crucial, such as in healthcare or social sciences.
However, manual video annotation comes with its own drawbacks. The process is time-consuming and can be labor-intensive, making it less scalable for large datasets. Additionally, factors like subjectivity can affect the consistency of the labels applied. Training annotators becomes essential to mitigate these issues, although it does not eliminate all potential biases. Organizations must weigh the trade-offs of this technique carefully, especially when dealing with significant amounts of data.
Automated Video Labeling Methods
Automated video labeling methods utilize algorithmic approaches, typically powered by artificial intelligence and machine learning. These methods are designed to process vast amounts of video data quickly and efficiently. Automated systems can analyze patterns, identify objects, and even predict contextual meanings from video frames, significantly reducing the time required for annotation.
While automation brings about greater scalability, it is not without its challenges. Accuracy in automated labeling can vary significantly based on the quality of the training data and the sophistication of the algorithms used. Mislabels in automated systems can lead to serious implications, especially in fields where precision is critical. Despite these risks, advancements in deep learning algorithms, like convolutional neural networks, continue to improve the reliability of automated methods.
Hybrid Approaches
Hybrid approaches combine the strengths of both manual and automated video labeling. In this method, an automated system performs the initial annotation, which is then refined and validated by human annotators. This setup aims to capitalize on the efficiency of automation while ensuring that the human oversight provides the necessary accuracy. The blend of these techniques leads to a reduction in overall costs and turnaround times without compromising on quality.
Nonetheless, hybrid approaches require careful planning and resource allocation. Human oversight is still needed to refine the automated labels, meaning organizations must invest in their workforce. An effective collaboration between technology and human insight is critical for achieving desired outcomes in video labeling. These approaches prove to be beneficial especially in domains where data quality is paramount.
In summary, the choice of video labeling technique profoundly affects the quality of data produced. By understanding manual, automated, and hybrid approaches, professionals can make informed decisions that align with their data objectives and project requirements. Effective implementation of these core techniques ensures that data is not just abundant but also valuable for driving insights.
Challenges in Video Labeling
The field of video labeling is marked by several challenges that significantly impact its efficiency and effectiveness. Understanding these challenges is crucial for any professional engaged in data annotation and machine learning. The complexities inherent in video data, including high volume and diverse content, require robust strategies to ensure that labels are accurate and reliable. This section addresses key challenges like scalability, the subjectivity involved in labeling, and the necessity for stringent quality control measures.
Scalability and Volume of Data
Scalability is a major concern when it comes to video labeling. With the rise of big data, the amount of video content generated is increasing exponentially. For instance, platforms like YouTube and TikTok upload hours of video content every minute, creating an overwhelming challenge for annotators. The sheer volume of data means that manual labeling can often become impractical and time-consuming.
Automated methods may mitigate some of these scalability issues, but they come with their own limitations. Thus, balancing scalability with high-quality outputs is essential. Companies must invest in scalable solutions while ensuring that the labeling process remains efficient and produces reliable results. Failure to address scalability could lead to bottlenecks in machine learning project timelines, negatively affecting productivity and outcomes.
Subjectivity in Labeling Decisions
Subjectivity poses another significant challenge in the realm of video labeling. Different annotators may interpret video content in distinct ways, leading to inconsistencies in labeling. For example, consider a video depicting a traffic incident. One annotator may label it as "accident," while another might categorize it as "distracted driving" due to their interpretation of the events. This variability makes it difficult to train machine learning models effectively.
To overcome this challenge, it's crucial to establish standardized guidelines for labeling. Clear criteria help reduce ambiguity and foster a more uniform approach to project tasks. However, no system is flawless; thus architects of labeling systems must implement regular reviews and feedback loops to further mitigate subjectivity.
Quality Control Measures
Quality control is paramount in the video labeling process. Without robust quality assurance methods, annotated data can become flawed and unreliable. The implications of poor-quality labels are significant; machine learning models built on faulty data can yield inaccurate predictions, ultimately compromising the integrity of the entire project.
Effective quality control measures include random spot-checks of completed annotations, peer reviews, and feedback mechanisms for annotators. Additionally, integrating technology such as AI-assisted review tools can enhance the quality of labeled data. By utilizing automated checks, organizations can quickly identify anomalies in labeling practices and rectify them accordingly.
"The quality of labeled data directly influences the effectiveness of machine learning applications across various sectors."
In summary, addressing the challenges of scalability, subjectivity, and quality control in video labeling is essential for maximizing the capabilities of machine learning models. By recognizing these challenges, professionals can implement strategic solutions that enhance the accuracy and reliability of video labeling efforts.
Applications of Video Labeling
Video labeling plays a crucial role in various sectors by enhancing the quality and applicability of video data for analysis. This process is not just about assigning tags or annotations; it involves a detailed understanding of the context within which the video is captured. The significance of video labeling arises from its ability to facilitate machine learning applications, leading to improved outcomes in autonomous systems, healthcare, security, and even sports analytics.
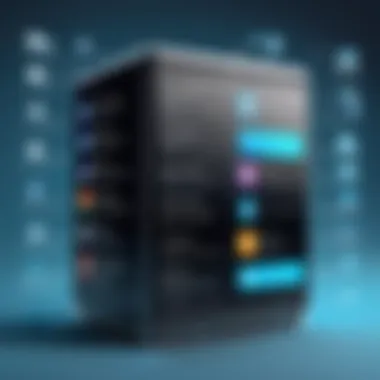
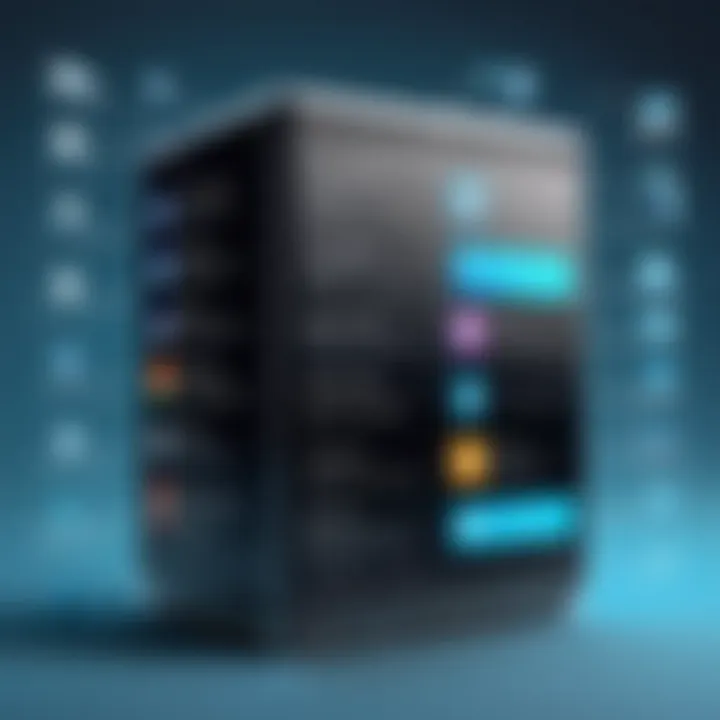
Key benefits of video labeling include:
- Data Precision: Accurate annotations lead to better machine learning models.
- Faster Decision Making: Automated insights derived from labeled data can expedite processes across industries.
- Enhanced Understanding: Detailed labeling helps create a clearer narrative around complex data, making it easier for professionals to draw actionable conclusions.
Considering these advantages, it is essential to explore how specific applications of video labeling operate within their respective fields.
In Autonomous Vehicles
The integration of video labeling in autonomous vehicles is transformative. Vehicles equipped with cameras rely on vast amounts of labeled video data to understand their surroundings. This data helps in identifying pedestrians, traffic signs, or other vehicles, enabling a safe navigation experience.
In essence, video labeling supports the development of intricate object detection algorithms, which are fundamental for the vehicle's decision-making process. As autonomous technology advances, the demand for high-quality labeled video will continue to grow, thereby improving safety and efficiency on roads.
In Healthcare and Medical Imaging
Video labeling in healthcare, particularly in fields like medical imaging, is vital for accurate diagnostics. Labeled video data can illuminate patterns in medical scans that may otherwise be missed. For instance, video annotations in surgical procedures assist in training algorithms for recognizing instruments or even anatomical structures. This application not only enhances the training of machine learning models but also fosters better patient outcomes through more precise surgical procedures.
In Security and Surveillance
In security and surveillance, video labeling is essential for threat detection and analysis. Security systems utilize labeled video data to monitor behaviors or identify suspicious activities. Machine learning models that are trained on such data can effectively distinguish between normal and abnormal activities, thus providing a strong line of defense against potential threats.
Accurate video labeling helps reduce false positives in security systems, ultimately saving resources and enhancing response times by alerting security personnel only when truly needed.
In Sports Analytics
Sports organizations are increasingly turning to video labeling for performance analysis and strategy optimization. Labeled videos of games allow analysts to quantify player movements, track ball possession, and assess team dynamics. These insights can significantly influence coaching decisions and team formations. In a competitive landscape, having accurate and detailed video data can be a game changer.
The labeling process in this context also helps in advancing fan engagement by providing deeper analysis that can be shared through various media channels.
Best Practices for Effective Video Labeling
Video labeling is an intricate process that demands attention to detail and consistency. Practicing effective video labeling establishes a framework for high-quality data annotation, which in turn enhances the performance of machine learning models. Adhering to best practices can elevate the standard of video annotation, paving the way for more accurate insights and automation.
Establishing Clear Labeling Guidelines
Clear labeling guidelines are fundamental to achieving quality in video annotation. These guidelines should define precisely what elements need to be labeled, the criteria for labeling, and the formats to be used. When annotators have a solid understanding of their responsibilities, they are more likely to produce consistent results.
Consider these points when developing guidelines:
- Specify Categories: Identify distinct labels required for different objects or scenes in the video. For instance, if labeling in healthcare videos, differentiate between types of medical equipment or actions.
- Consistent Format: Use a standard format for labeling. This aids in integrating the labeled data into machine learning frameworks without errors.
- Examples and Non-Examples: Provide concrete examples and non-examples of each category. This practice helps annotators grasp the boundaries of each label more effectively.
By following these steps, organizations can minimize the subjectivity often found in the manual labeling process.
Utilizing Reliable Tools and Software
Selecting the right tools and software is key to efficient video labeling. Reliable annotation software not only maximizes productivity but also minimizes the chances of errors in labeling. It is essential to choose tools that fit the specific needs of the labeling task.
Here are some recommendations when evaluating tools:
- User-Friendly Interface: The software should have an intuitive design. Annotators will work more efficiently if they can navigate the software easily.
- Collaboration Features: Consider tools that allow multiple users to annotate simultaneously, as this can speed up the labeling process significantly.
- Integration Capabilities: Look for software that easily integrates with data storage solutions and frameworks for machine learning. Seamless integration can save time during pre-processing.
Continuous Training for Annotators
Training is critical for maintaining the quality and consistency of video labeling. Human annotators may have varying levels of understanding and skill sets, which can lead to discrepancies in the labeling process. Continuous training helps in bridging that gap.
Important aspects of training include:
- Regular Workshops: Conducting workshops focused on specific aspects of labeling can help keep annotators updated about best practices and recent tools.
- Feedback Mechanism: Implement a system for providing constructive feedback to annotators. This allows them to learn from their mistakes and improve over time.
- Skill Assessment: Carry out periodic assessments to evaluate the skills of annotators. Regular assessments ensure that the team remains competent in their labeling tasks.
By investing in the continuous training of annotators, organizations can improve the quality of their outputs and the reliability of their machine learning models.
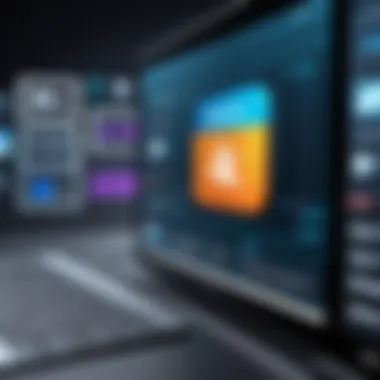
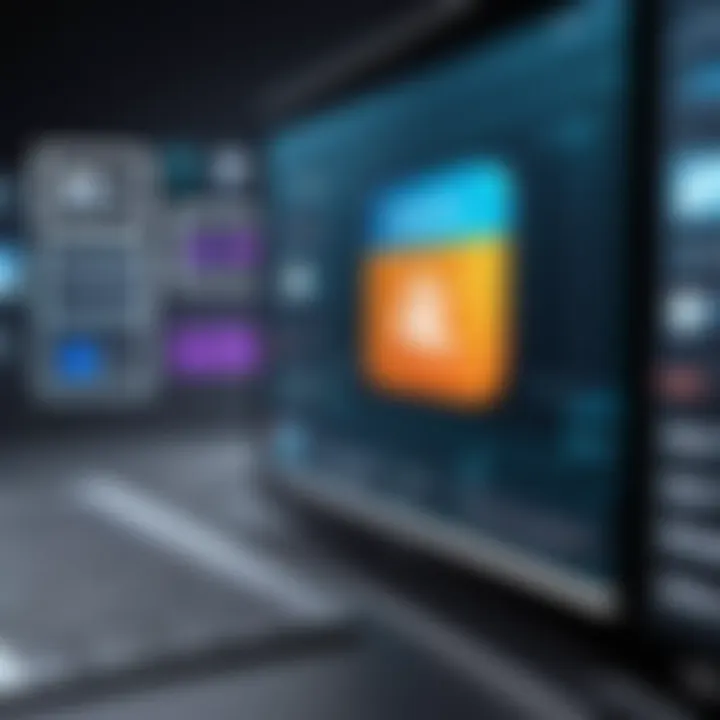
"Quality video labeling is not just about adding labels; it's a meticulous process that shapes the future of machine learning and AI applications."
In summary, implementing best practices in video labeling, including clear guidelines, reliable tools, and continuous training, significantly enhances the quality and efficiency of the labeling process. This ultimately leads to richer datasets and more accurate outputs across various applications.
The Role of Technology in Video Labeling
The integration of technology into video labeling is not merely supplementary; it is fundamental. In todayโs data-driven landscape, the demand for effective and efficient methods of video labeling has surged. As machine learning models require vast amounts of data, how this data is labeled can significantly influence model accuracy. Consequently, understanding the role of technology in this domain cannot be overstated. It encompasses both the tools available for video annotation and the methodologies adopted to enhance the labeling process.
Artificial Intelligence and Machine Learning Integration
Artificial intelligence (AI) and machine learning (ML) are at the forefront of advancements in video labeling. Their ability to automate and improve the labeling process has been a game-changer for numerous industries. Using algorithms to analyze video content, systems can identify and label objects, actions, and events without extensive human intervention. This capability not only reduces the time taken for annotation but also increases consistency and scalability.
For instance, convolutional neural networks (CNNs) have shown remarkable success in image and video analysis. They process data in a way that mimics human visual perception. By training on labeled datasets, these networks learn to recognize patterns and can thus autonomously label new footage. The implications of AI in video labeling stretch across various sectors. Deploying these technologies leads to:
- Increased Speed: The manual labeling process can be time-consuming. AI reduces this time significantly.
- Scalability: As the volume of data grows, AI systems maintain efficiency without needing a proportional increase in human labor.
- Improved Accuracy: AI minimizes human error, offering more reliable labels.
However, it is crucial to recognize that the success of AI in video labeling depends heavily on the quality and breadth of the training datasets. If the training data is biased or insufficient, the models may produce flawed results.
Cloud-Based Video Annotation Platforms
The advent of cloud-based video annotation platforms has transformed how teams approach video labeling. These platforms facilitate remote collaboration among various stakeholders, making it easier to manage projects that require input from multiple experts. Additionally, they provide scalable storage solutions, essential for handling large video datasets.
Such platforms typically offer various features to streamline the annotation process:
- Intuitive Interfaces: User-friendly designs allow annotators to focus on labeling without extensive training.
- Real-time Collaboration: Multiple users can work on the same project simultaneously, improving efficiency.
- Integration with AI Tools: Many of these platforms now incorporate AI capabilities to assist annotators, providing suggestions or automated labels that humans can then verify.
The benefits of using cloud-based annotation platforms extend to data security and accessibility. Since the data is stored in the cloud, it can be accessed from anywhere, provided there is an internet connection. This flexibility supports teams working in diverse locations and encourages a more agile workflow.
Future Trends in Video Labeling
Video labeling is rapidly transforming, driven by innovations in technology and user needs. Understanding future trends helps businesses and researchers prepare for the changes that will shape video annotation practices. These trends promise to enhance efficiency, accuracy, and user experience across many sectors.
Advancements in Deep Learning Algorithms
One of the most significant trends lies in the advancements of deep learning algorithms. These algorithms are powering automated video labeling systems, allowing for much faster processing of large datasets. Complex models like convolutional neural networks (CNNs) are becoming standard in recognizing patterns and objects within videos.
These improvements contribute to more reliable predictions and classifications, reducing the need for manual intervention. However, it is important to note that the effectiveness of these algorithms depends on the quality of the labeled data used to train them. Therefore, while deep learning continues to evolve, it is crucial to maintain rigorous labeling standards to fine-tune these models.
"Deep learning algorithms represent a leap in accuracy and speed but come with a dependence on well-labeled datasets for training and validation."
Evolution of User Experience in Labeling Tools
As video annotation becomes more integral to data processing, the evolution of user experience in labeling tools has gained prominence. Tools are being redesigned to be more intuitive and accessible for annotators. Features like drag-and-drop interfaces and advanced visualization techniques streamline the labeling process.
Additionally, collaborative tools are on the rise. They allow multiple users to annotate videos simultaneously, improving the overall efficiency of the labeling task.
The incorporation of feedback mechanisms is also an emerging trend. Annotators can receive real-time suggestions or corrections, increasing accuracy and user satisfaction. Companies that recognize the importance of user experience in their labeling tools are likely to see better outcomes in data quality and productivity.
Closure
Summary of Key Takeaways
To summarize, the main points discussed in this article include:
- Definition and Importance: Video labeling is essential for training machine learning models by providing annotated data.
- Techniques: The article highlighted the distinctions between manual annotation, automated systems, and hybrid approaches.
- Challenges: Issues such as scalability, subjectivity, and quality control can complicate the labeling process.
- Applications: The use of video labeling spans multiple industries, from autonomous vehicles to healthcare and sports analytics.
- Best Practices: Establishing clear guidelines, using reliable tools, and ensuring continuous training for annotators are crucial for successful video labeling.
- Future Trends: Advancements in deep learning and user experience in labeling technologies point to a promising future for this field.
The Importance of Video Labeling in Data Processing
Video labeling plays an underrated yet vital role in the overall data processing landscape. It provides a foundation upon which algorithms can learn and improve their predictive accuracy. High-quality labeled data becomes increasingly essential as artificial intelligence solutions proliferate in various industries.
By investing in effective video labeling, organizations can realize numerous benefits, including:
- Improved accuracy in machine learning models
- Increased operational efficiency through automation
- Better analytical insights, leading to enhanced decision-making
- Greater customer satisfaction through improved products and services
In summary, the significance of video labeling cannot be overstated. Recognizing its value in data processing leads to more informed strategies in technology deployment and showcases its importance as a driver of innovation.