Understanding Stat Analytics: Key Insights and Applications
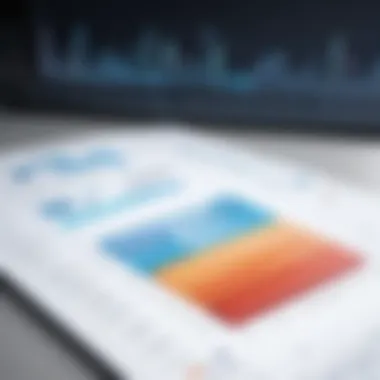
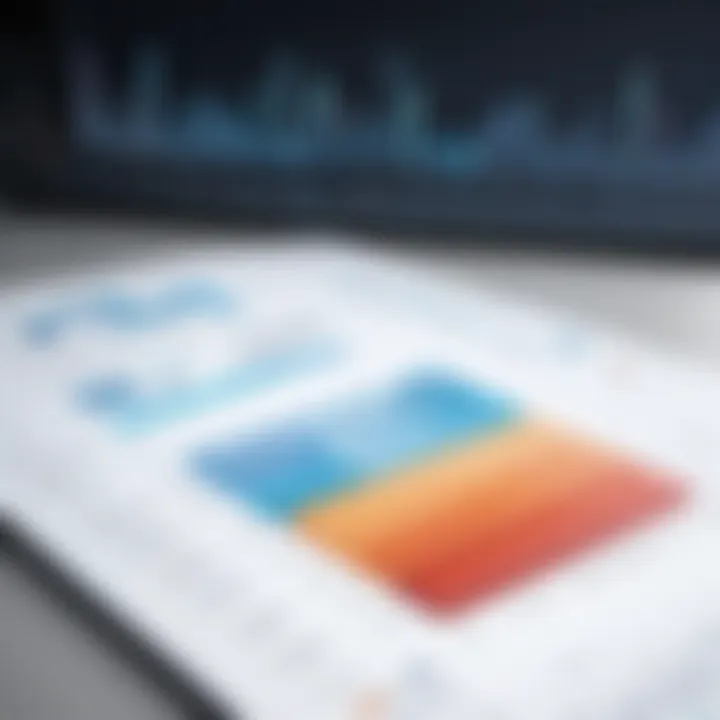
Intro
Stat analytics has become a crucial component in modern decision-making processes. In a world inundated with data, the ability to extract meaningful insights can differentiate between success and failure in various industries. This article aims to provide a thoughtful examination of stat analytics, covering its principles, applications, and the ethical considerations that surround it. A strong foundation in statistical methods and tools will aid professionals in making informed choices based on the data at hand.
Software Needs Assessment
For organizations to effectively engage in stat analytics, a thorough software needs assessment is critical. This evaluation ensures that the tools and technologies utilized align with the specific analytical requirements of the users.
Identifying User Requirements
Understanding user requirements involves interacting with stakeholders to gain insight into their preferences and analytic objectives. This includes factors like:
- The types of data they work with.
- The complexity of analyses they are undertaking.
- The level of expertise possessed by users.
- Desired output formats and reporting capabilities.
The clarity gained from identifying these requirements dictates the subsequent steps in selecting software solutions.
Evaluating Current Software Solutions
Once user needs have been established, it is important to evaluate existing software options. This process involves:
- Researching available software, such as R, Python, or Tableau, that can satisfy analytic goals.
- Comparing functionality and features, examining aspects like visualization capabilities, data handling, and integration with other systems.
- Reading user reviews and case studies to assess real-world performance and applicability.
Evaluation should culminate in a decision matrix, which outlines how well each potential software solution meets identified requirements, thus facilitating an informed choice.
Data-Driven Insights
In stat analytics, drawing insights from data requires a robust understanding of market trends and performance metrics. These elements guide strategic initiatives and illustrate how analytics drive decision-making.
Market Trends Overview
Staying current with market trends can reveal patterns that affect performance. Key trending aspects include:
- Emerging technologies transforming data interpretation.
- Consumer behavior shifts influencing business strategies.
- Regulatory changes impacting data usage and governance.
Engagement with these evolving trends ensures organizations remain competitive and proactive.
Performance Metrics
Metrics play a pivotal role in evaluating data effectiveness. Common performance metrics include:
- Return on Investment (ROI): An essential measure determining the profitability of data initiatives.
- Customer Lifetime Value (CLV): Understanding the long-term value of customers can shape marketing and sales strategies.
- Churn Rate: Monitoring this rate helps identify areas for retention improvements.
"Understanding performance metrics is essential for making informed decisions in stat analytics. It defines success and guides future actions."
Conclusively, the essence of stat analytics lies in its systematic approach to data analysis. By focusing on software needs, user requirements, market trends, and performance metrics, professionals can better navigate the complexities of data-driven decision-making. Through careful assessment and strategic implementation, organizations can harness the power of stat analytics to drive their success.
Prelude to Stat Analytics
Stat analytics plays a crucial role in today’s data-driven landscape. It enables organizations to make informed decisions by leveraging data insights. Understanding this field is essential for professionals across various sectors, as it bridges the gap between raw data and actionable insights. Through structured methods and robust analysis, stat analytics transforms complexities into comprehensible information, fostering data-driven cultures.
Defining Stat Analytics
Stat analytics refers to the systematic application of statistical methods to analyze data. It involves collecting, examining, interpreting, and presenting data to uncover patterns and trends. The primary aim is to derive meaningful insights that guide decision-making processes. The field encompasses a variety of techniques, each tailored to specific data types and business needs.
To define stat analytics more profoundly, it integrates tools such as regression analysis, hypothesis testing, and data visualization. Stat analytics uses descriptive statistics to summarize data sets while employing inferential statistics to make predictions about larger populations based on sample data. This duality allows an organization to not only understand its data contextually but also to project future outcomes effectively.
Historical Context
The roots of statistical analysis can be traced back centuries. Many foundational principles emerged during the 18th and 19th centuries, defined by the works of pioneers like Karl Pearson and Ronald Fisher. These early contributions laid the groundwork for modern statistics.
Over time, the evolution of technology has radically transformed how data is collected and analyzed. Once data was largely qualitative and collected manually, it has now shifted to quantitative and automated methods, allowing for faster processing and larger volumes of data. The rise of computing power and software has further accelerated advancements in stat analytics. Today, organizations rely on powerful statistical software to analyze data and derive actionable insights in real-time.
In summary, understanding the historical context of stat analytics provides insight into its significance in contemporary analysis. It underlines the rigorous methods developed over time, showcasing the importance of data analysis in various domains.
Core Principles of Statistical Analysis
Statistical analysis forms the backbone of effective data-driven decision making. Recognizing the core principles of statistical analysis is crucial for practitioners across various fields. These principles guide the interpretation and extraction of insights from data. By understanding these foundational concepts, professionals can better deploy statistical techniques in their respective domains, enhancing accuracy and effectiveness in their analyses.
Descriptive vs. Inferential Statistics
Descriptive statistics focus on summarizing and describing the main features of a data set. This includes measures such as mean, median, mode, and standard deviation, which provide insight into the central tendency and variability of the data. These statistics are vital for understanding the basic characteristics of the dataset without making predictions or generalizations beyond the observed data.
On the other hand, inferential statistics allow us to make predictions and generalizations about a population based on a sample of data. Techniques such as hypothesis testing, confidence intervals, and regression analysis fall under this category. Inferential statistics let researchers draw conclusions and make informed decisions with a quantifiable level of uncertainty. Thus, the distinction between these two branches is critical. Descriptive statistics lay the groundwork, while inferential statistics extend findings beyond mere observations.
Probability Theory
Probability theory is a core element of statistical analysis. It provides the foundational framework for understanding random events and the likelihood of various outcomes. This theory allows analysts to model uncertainty and quantify risk, which is essential in fields like finance and healthcare.
Key concepts in probability include random variables, probability distributions, and independent events. An understanding of these concepts enables statisticians to predict future occurrences or behaviors based on past data. For instance, probability distributions inform decision-makers about how values are expected to disperse over time or within a given scenario. Thus, mastering probability theory equips analysts with tools to evaluate risks and benefits effectively.
Sampling Techniques
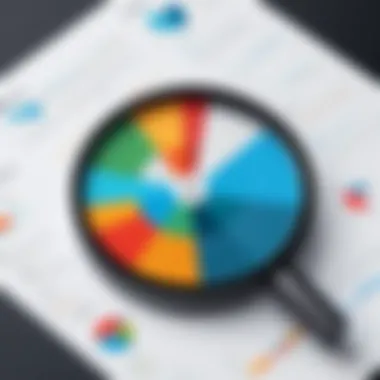
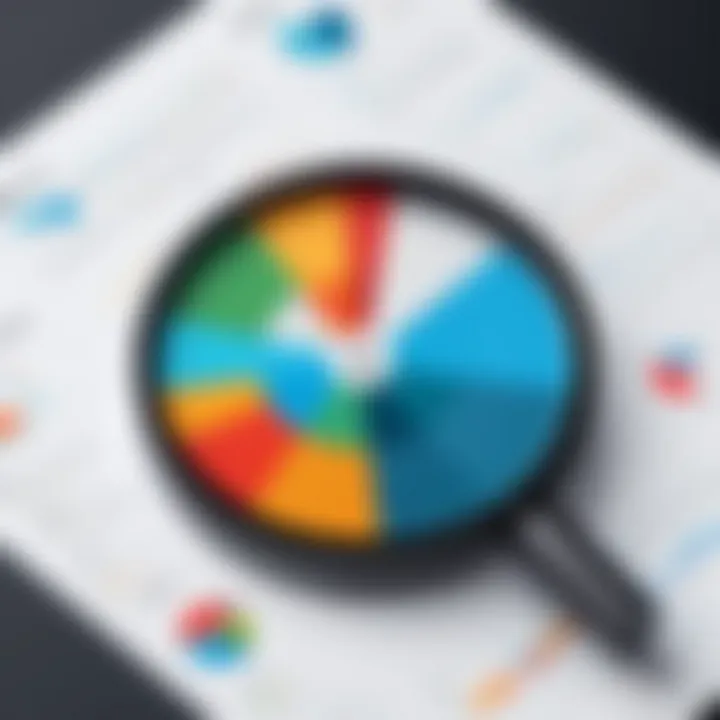
Sampling techniques play a pivotal role in the accuracy and reliability of statistical analyses. These techniques determine how data is collected and the representativeness of the sample used. Proper sampling methods ensure that results can be generalized to the larger population without bias.
There are several types of sampling techniques:
- Random Sampling: Each member has an equal chance of selection, minimizing bias.
- Stratified Sampling: The population is divided into distinct subgroups, and samples are taken from each group to ensure representation.
- Cluster Sampling: Entire clusters or groups are sampled, often used for practical reasons in large populations.
Understanding and employing the right sampling techniques is essential to achieve valid statistical inferences and insights. Incorrect sampling can lead to skewed results, thereby undermining the overall analysis.
"Statistical methods, when correctly applied, uncover truths in data which might otherwise remain hidden."
In summary, a solid grasp of the core principles of statistical analysis is indispensable for any professional involved with data. Descriptive and inferential statistics provide the framework for understanding and generalizing data. Probability theory enhances risk assessment, while effective sampling techniques ensure accuracy. All these elements synergize to inform better decision-making in various fields.
Applications of Stat Analytics
Stat analytics holds significant importance across various industries. By harnessing statistical methods, organizations can glean insights from their data, leading to informed decision-making and strategic advantages. The applications of stat analytics are numerous, each offering unique benefits that cater to specific business needs. This section explores the key areas where stat analytics plays a crucial role, emphasizing how they can enhance operational efficiency, customer satisfaction, and overall performance.
Business Intelligence
Business Intelligence (BI) is a critical area that leverages stat analytics to turn raw data into actionable insights. By analyzing data from various sources, companies can examine trends, identify opportunities, and make data-driven decisions. Tools like Power BI and Tableau are commonly used to visualize these insights.
Here are some key benefits of applying stat analytics in business intelligence:
- Enhanced Decision-Making: Analytical insights guide management in choosing strategies that align with organizational goals.
- Performance Monitoring: KPIs can be tracked effectively to assess the progress and make necessary adjustments.
- Market Understanding: Businesses can analyze customer behavior and market trends, helping them to target advertising efforts better.
Healthcare Analytics
Healthcare analytics is another area where stat analytics shines. The healthcare industry generates vast amounts of data, from patient records to treatment outcomes. Stat analytics can improve patient care by predicting health trends and identifying at-risk populations.
Some notable applications include:
- Predictive Modeling: Forecasting patient admissions allows for better resource allocation.
- Outcome Analysis: Evaluating treatment effectiveness contributes to improved patient care protocols.
- Cost Reduction: Identifying inefficiencies can lead to significant cost savings for healthcare providers.
Financial Analytics
The financial sector is heavily reliant on stat analytics for risk assessment and investment analysis. By employing statistical methods, financial institutions can evaluate portfolio performance and financial risks with greater accuracy.
Key aspects of financial analytics include:
- Risk Management: Statistical models assess the likelihood of default on loans or investments.
- Fraud Detection: Identifying unusual patterns can help prevent financial fraud.
- Valuation Models: Analyzing market data to predict stock prices enhances investment strategies.
Marketing Analytics
In the realm of marketing, stat analytics offers invaluable support in understanding customer preferences and measuring campaign effectiveness. By utilizing analytic tools, marketers can refine their strategies and improve ROI.
Key benefits consist of:
- Customer Segmentation: Analyzing customer data allows for targeted marketing strategies, improving engagement.
- Campaign Effectiveness: Measuring the return on investment of marketing campaigns helps in resource allocation.
- Market Trend Analysis: Continuous monitoring of market and consumer behavior keeps businesses ahead of the competition.
"In a world increasingly driven by data, the application of stat analytics is no longer optional; it is essential for sustainable success across industries."
Applications of stat analytics provide organizations with the necessary insights to drive growth. In the competitive landscape of modern business, leveraging statistical methods enhances the ability to operate efficiently while addressing the specific needs of customers and the market.
Tools and Technologies for Stat Analytics
Understanding the tools and technologies used in stat analytics is crucial. They serve as the backbone for gathering, analyzing, and visualizing data. With rapid advancements in technology, these tools allow professional to extract actionable insights more efficiently and accurately. Choosing the right tools can impact not just the speed of analysis but also the quality of the insights derived. In sectors where data is abundant, leveraging these technologies is not just beneficial, it's essential.
Statistical Software Packages
Statistical software packages are widely used in the field of stat analytics. Some of the most common tools include SAS, SPSS, R, and Python’s libraries like Pandas and NumPy. These packages offer a wide range of statistical methods and procedures that are fundamental for detailed data analysis.
One advantage of using these software packages is their ability to handle large data sets. For instance, R is particularly strong in statistical modeling and visualization. SAS is favored in corporate settings for its robust data management capabilities. Moreover, Python's libraries provide flexibility for data manipulation and analysis.
When selecting a statistical software package, consider the following:
- Ease of use: Some packages are more user-friendly while others require programming skills.
- Cost: Open-source software like R is free, whereas packages like SAS may come at a premium.
- Community support: A large community can provide additional resources and support. Both R and Python have strong communities to assist users.
Data Visualization Tools
Data visualization is essential in stat analytics. It helps to distill complex datasets into clear and understandable visuals. Tools like Tableau, Power BI, and Microsoft Excel are frequently utilized. These tools enable users to create interactive dashboards and reports, making data insights accessible to stakeholders.
The importance of data visualization lies in its ability to reveal trends, patterns, and outliers that might not be apparent in raw data. For example, Tableau allows for real-time data visualization, facilitating prompt decision-making.
Factors to consider when choosing a data visualization tool include:
- Interactivity: Tools should allow users to interact with the data dynamically.
- Integration: The ability to integrate with other data sources and software is crucial for seamless analysis.
- User interface: A straightforward user interface enhances usability, making it easier for teams to adopt.
Programming Languages in Statistics
Programming languages like R and Python play a pivotal role in stat analytics. They provide capabilities that are necessary for performing complex analyses. R is specifically designed for statistical computing, making it highly specialized. On the other hand, Python offers broader software development versatility while still being equipped with powerful libraries for data analysis.
Utilizing programming languages allows analysts to:
- Write custom scripts for specific analyses.
- Automate repetitive tasks, thus improving efficiency.
- Access a variety of libraries that extend functionality, such as Scikit-learn for machine learning.
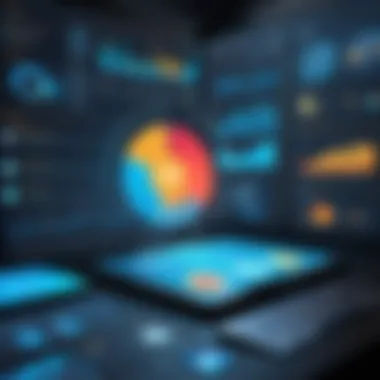
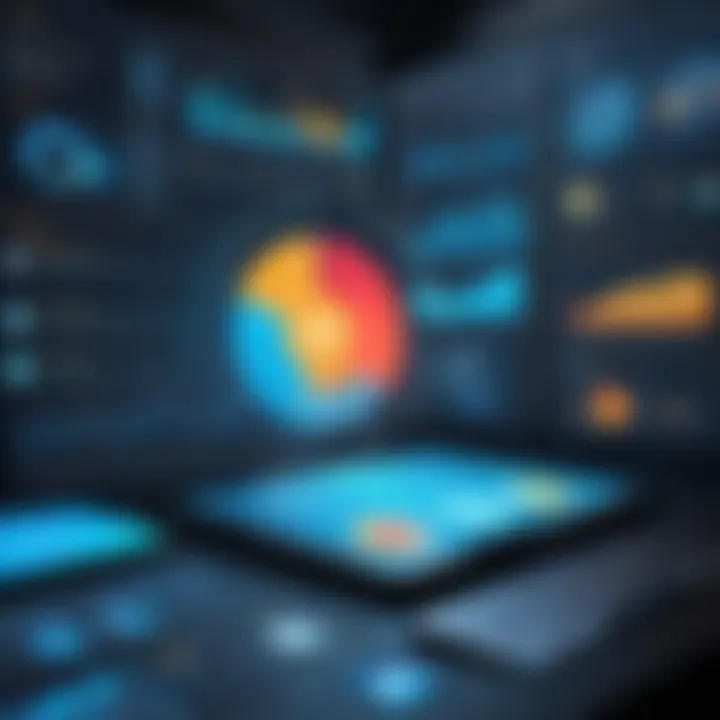
Methodologies in Stat Analytics
In the field of stat analytics, methodologies play a crucial role. They define the framework through which data is collected, analyzed, and interpreted. Effective methodologies ensure the accuracy of insights drawn from data, which helps inform decision-making processes. Each method has its own unique benefits and considerations that must be understood to apply them effectively.
Regression Analysis
Regression analysis is a statistical method used to examine the relationship between two or more variables. It provides not just a way to assess whether there is a correlation, but also to make predictions based on data trends. By understanding how independent variables impact a dependent variable, analysts can gauge potential outcomes under different scenarios.
- Types of Regression: There are various forms of regression analysis, including linear regression, logistic regression, and multiple regression. Each type addresses specific data challenges and scenarios.
- Applications: Businesses often use regression for forecasting sales, evaluating marketing strategies, and understanding customer behavior.
"Understanding regression analysis equips professionals with the tools to predict future trends based on historical data."
Hypothesis Testing
Hypothesis testing is a method used to determine the validity of a statement or assumption regarding a parameter in a dataset. This method provides a systematic way to test assumptions and make informed conclusions. It essentially helps validate or refute hypotheses through statistical evidence.
- Key Components: The testing process involves defining null and alternative hypotheses, selecting a significance level, and calculating a p-value to determine whether to reject the null hypothesis.
- Importance: In business, hypothesis testing can be important for evaluating the effectiveness of a marketing campaign or a new product feature based on customer feedback or sales data.
Time Series Analysis
Time series analysis involves statistical techniques used to analyze time-ordered data points. This method assesses past trends to forecast future movements. It is particularly useful in fields like finance and economics, where decision-making relies heavily on past performance and seasonal variations.
- Components: Time series analysis includes decomposition into trends, seasonal patterns, and irregular components.
- Use Cases: It is commonly applied in stock price forecasting, economic indicator analysis, and demand forecasting.
By employing these methodologies, professionals in stat analytics can derive valuable insights from complex datasets. Their correct application is essential for making data-driven decisions in various fields.
Data Quality and Its Impact on Stat Analytics
Data quality plays a critical role in the realm of stat analytics. The integrity of data directly influences the outcomes of analyses and the decisions based on those analyses. If the data is flawed, the insights drawn from it will likely be misleading. Thus, ensuring high data quality is not merely advisable; it is essential for effective decision-making.
In this section, we will discuss two core components related to data quality: the importance of data cleaning and the techniques used for data validation. Understanding these elements helps professionals grasp the larger implications of data quality and how it affects their operational success.
Importance of Data Cleaning
Data cleaning involves identifying and correcting errors in the data set. This step is crucial as it ensures the data is accurate, complete, and relevant. For example, inaccuracies can arise from various sources, such as human error in data entry, outdated information, or inconsistencies in data formatting.
The benefits of data cleaning include:
- Enhanced Accuracy: High-quality data leads to accurate analyses, which in turn fosters better decision-making.
- Improved Decision-Making: Reliable insights allow organizations to strategize based on facts rather than assumptions.
- Time Efficiency: Clean data can reduce the time spent on troubleshooting errors in analysis.
- Regulatory Compliance: Many industries have regulations that demand high data quality; thus, cleaning processes help organizations adhere to these standards.
Consequently, investing time and resources in data cleaning can yield significant returns for any analytics initiative.
Data Validation Techniques
Data validation ensures that the data being processed meets specific quality standards and is fit for use. Various techniques are employed to validate data, providing a framework to assess data quality. Here are some commonly used methods:
- Range Checks: This technique examines whether data values fall within defined limits. For example, age data should not contain negative values.
- Format Checks: This involves ensuring data adheres to a specific format. Email addresses, for instance, should conform to standard email formatting.
- Consistency Checks: These ensure that data does not conflict with other information in a dataset. For example, if a customer is marked as "young" in one dataset but "senior" in another, a consistency check will flag it.
- Uniqueness Checks: This validates that data entries do not duplicate where it shouldn't. Typically used in databases to ensure unique identifiers.
In summary, effective validation techniques can significantly enhance the reliability of analytics outputs. Without rigorous validation practices, results may not only be unreliable but also misleading.
"Data quality is not an act, it is a habit." – Author Unknown
By prioritizing data quality through cleaning and validation, organizations can harness the full power of stat analytics, making informed decisions that reflect the actual circumstances, and ultimately leading to greater operational success.
Ethical Considerations in Stat Analytics
As data-driven decision-making continues to thrive across various industries, the ethical considerations in stat analytics hold a crucial position. The impact of analytics on privacy, bias, and fairness cannot be understated. Ethical practices foster trust and credibility, allowing organizations to leverage data while respecting individual rights. This section will explore two primary aspects of ethics in analytics: data privacy issues and bias in data analysis.
Data Privacy Issues
Data privacy is a vital concern as organizations collect, store, and process vast amounts of personal information. In the realm of stat analytics, it is essential to understand how data is used and protected. There are several key aspects to consider:
- Informed Consent: Individuals should be informed about the purpose of data collection and how it will be used. This transparency is fundamental.
- Data Anonymization: Removing personally identifiable information can help mitigate risks. However, even anonymized data can sometimes be re-identified. Therefore, careful techniques must be employed to safeguard user privacy.
- Regulatory Compliance: Organizations must adhere to regulations such as the General Data Protection Regulation (GDPR) or the California Consumer Privacy Act (CCPA). Understanding and implementing these laws is critical to ethical data practices.
"Data privacy matters are not just legal obligations; they are ethical imperatives that affect individual rights and societal trust."
Bias and Fairness in Data Analysis
Bias in data analysis can significantly impact the interpretations made from statistical findings. Ensuring fairness is vital for the integrity of analytical outcomes. Some significant points include:
- Representation in Data: The datasets used must represent the diversity of the population. Underrepresentation can lead to skewed conclusions.
- Algorithmic Bias: Automated systems can perpetuate existing biases. Continuous monitoring and updates are necessary to mitigate such biases.
- Outcome Fairness: The results of statistical analyses should provide equitable outcomes for all demographics involved. Analyzing the methods used in data collection and interpretation is essential to identify potential biases.
In summary, ethical considerations in stat analytics are not just legal obligations but fundamental aspects that affect individual rights and public trust in data practices. Addressing data privacy issues and biases in analysis ensures credibility and effectiveness in statistical insights.
Challenges in Stat Analytics
Understanding the challenges involved in stat analytics is essential for any professional who wishes to effectively glean insights from data. Analytics is a powerful tool that can drive decision-making. However, it hinges on the quality of data and the ability to interpret complex information. This section will explore some key challenges faced in this field and underline their importance.
Interpreting Complex Data Sets
The interpretation of complex data sets is one of the most significant hurdles in stat analytics. Data often comes from diverse sources, and its structure can vary greatly. Hence, it requires skilled personnel to analyze it correctly. Two prominent factors must be considered here: data dimensionality and the sheer volume of data.
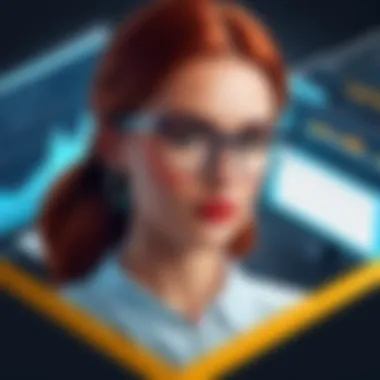
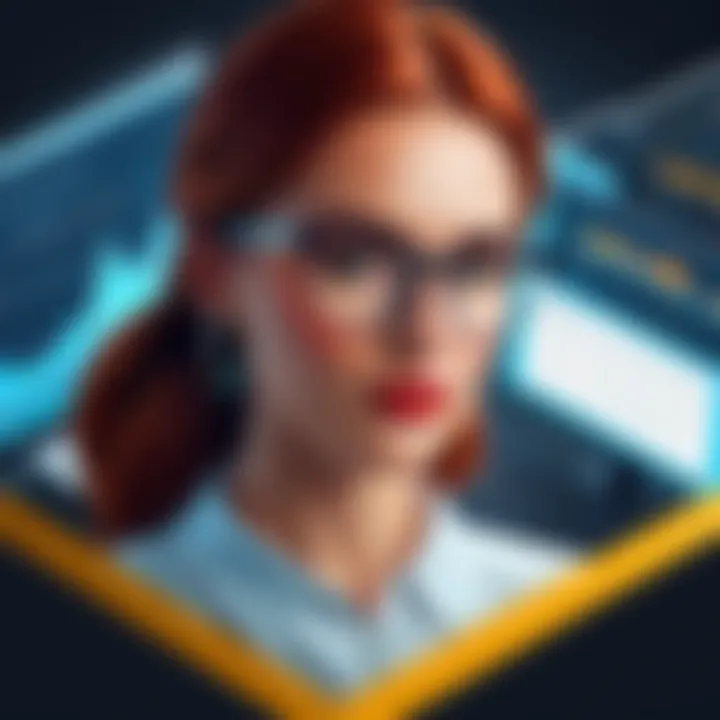
- Data Dimensionality: Complex data sets often involve multiple variables that interact with one another. Understanding these relationships is crucial. High dimensionality can lead to what is known as the "curse of dimensionality," making patterns difficult to discern. Specialized techniques such as dimensionality reduction or advanced visualizations are often necessary.
- Volume of Data: The amount of data generated today is immense. Big data technologies, such as Hadoop and Spark, enable the storage and processing of such vast quantities. However, interpreting this data efficiently often requires advanced statistical methods and algorithms. Misinterpretation can lead to incorrect conclusions or misguided strategies.
Analyzing complex data sets calls for a methodical approach. Utilizing robust statistical techniques and sophisticated data visualization tools can aid in this effort. Data scientists and analysts must enhance their skills continuously to keep pace with evolving methodologies in this area.
Adapting to Rapid Technological Changes
Rapid technological advancements present another challenge in the field of stat analytics. The arrival of new instruments, frameworks, and methodologies requires those in the analytics field to remain updated with current trends and tools. This continuous learning is not optional but rather essential to leverage the latest techniques effectively.
- Emergence of New Tools: New software and tools frequently emerge, such as Python libraries like Pandas and TensorFlow. Analysts must become proficient in these tools to remain competitive. The rate of change can often feel overwhelming, but staying informed through online courses or professional networks is crucial.
- Integration of Emerging Technologies: Technologies like machine learning and artificial intelligence fundamentally alter how data is analyzed. Techniques that were considered state-of-the-art just a few years ago may quickly become obsolete. It’s vital that analytics professionals not only learn these technologies but also understand how to integrate them into their existing frameworks.
"Technological changes in stat analytics demand a mindset of lifelong learning. An adaptable and flexible approach is fundamental in overcoming these challenges."
Ultimately, the ability to adapt to these rapid changes can determine the success of analytics projects. Having a growth mindset and focusing on continued education are vital for navigating the challenges in stat analytics.
Future Trends in Stat Analytics
In the rapidly evolving field of data analysis, understanding future trends is crucial for professionals aiming to maintain a competitive edge. Organizations are increasingly relying on data-driven decision-making, making advancements in statistical analytics pivotal. The following trends will shape how stat analytics will be implemented and utilized in various sectors.
Artificial Intelligence Integration
The integration of artificial intelligence (AI) in stat analytics represents a significant leap forward. AI technologies can enhance traditional statistical methods by improving the speed and accuracy of data analysis. For instance, machine learning algorithms can process vast amounts of data, identify patterns, and make predictions, all without the need for human intervention. This automation allows analysts to focus on interpreting results and making strategic decisions. The use of AI also helps in handling unstructured data, which is increasingly common in today's digital landscape. Moreover, AI can adapt and learn from new data inputs, ensuring that analytical models remain relevant and effective.
Real-time Data Processing
Real-time data processing is another trend gaining traction in stat analytics. Organizations now demand timely insights that can inform immediate decision-making. With the capability to analyze and interpret data as it is generated, businesses can respond quickly to market changes, customer behaviors, and other critical factors. This trend is enabled by advancements in cloud computing and data streaming technologies, allowing companies to harness data in real-time.
Adopting real-time data processing techniques offers several advantages:
- Swift Decision-making: Businesses can make informed decisions faster, gaining a competitive advantage.
- Improved Customer Interaction: Companies can personalize customer experiences by responding to needs immediately.
- Operational Efficiency: Reduces the time spent on data lag, streamlining processes and enhancing productivity.
"The integration of future trends will redefine how analytics shape business strategies and operations."
Case Studies of Successful Stat Analytics Implementations
In the realm of data analysis, case studies serve as instrumental narratives that showcase real-world applications of statistical analytics. They illuminate how organizations leverage data-driven strategies to make informed decisions, enhance operational efficiency, and drive growth. By examining both successful and unsuccessful uses of stat analytics, professionals can gain valuable insights into the complexities of implementation. These studies highlight processes, outcomes, and the lessons learned, thus offering a comprehensive understanding of best practices in stat analytics.
Business Case: Retail Sector
The retail sector extensively utilizes stat analytics to optimize various aspects of business operations. For instance, companies like Amazon employ advanced analytics to manage inventory effectively. By analyzing purchase patterns and seasonal trends, they can predict demand fluctuations. This strategy reduces the risk of overstock and stockouts, ultimately lowering operational costs and improving customer satisfaction.
- Customer segmentation is another critical element. Retailers use statistical methods to divide their customer base into distinct groups based on purchasing behavior. This targeted approach allows for personalized marketing campaigns and tailored promotions.
- The implementation of A/B testing provides insights into customer preferences. By analyzing different versions of product displays or marketing messages, retailers can determine which approach yields the best results in terms of sales conversion.
Effective case studies in the retail sector demonstrate the transformative effect of data analytics in shaping consumer experiences and optimizing logistical operations.
Healthcare Case: Patient Outcome Improvement
In healthcare, stat analytics plays a vital role in improving patient outcomes. Hospitals employ statistical methods to track and analyze patient data, which can inform clinical decisions and streamline care protocols. For example, the Cleveland Clinic adopted predictive modeling to identify patients at high risk of readmission. This analytical approach helped healthcare providers to implement preventive strategies, reducing readmission rates significantly.
- Disease trend analysis enables healthcare professionals to monitor outbreaks and allocate resources effectively. By analyzing historical data, they can identify patterns in disease progression and inform public health interventions.
- The integration of electronic health records with analytics tools enhances patient care. This data amalgamation allows practitioners to analyze treatment effectiveness for diverse demographics, thus refining their treatment plans.
Best Practices in Conducting Stat Analytics
Effective stat analytics requires adherence to established best practices. These practices ensure the reliability and validity of the results, guiding professionals in making sound decisions based on data. The importance of implementing best practices goes beyond merely following established protocols; they foster a culture of rigorous analytical thinking, ultimately enhancing project outcomes.
Establishing Clear Objectives
When embarking on any statistical analysis, it is crucial to first define clear objectives. This step provides direction throughout the analytics process. Objectives should be specific, measurable, achievable, relevant, and time-bound. Clear objectives help in three main ways:
- Focus: It aligns the team’s efforts, ensuring everyone works towards a common goal.
- Measurement: Objectives serve as benchmarks for success, allowing for assessment and evaluation of the analysis effectiveness.
- Relevance: They help determine which data points and analytical methods are necessary, enhancing the efficiency of the process.
With clear goals, professionals can utilize appropriate techniques and tools to extract insights relevant to their inquiries.
Collaborating Across Disciplines
Collaboration is essential in stat analytics. Engaging diverse disciplines enriches the analytical process by incorporating various perspectives and expertise. This multidisciplinary approach can lead to several benefits:
- Enhanced problem-solving: Different viewpoints can illuminate potential biases or blind spots.
- Broader knowledge base: Team members bring specialized skills in areas like data science, domain knowledge, and statistical methodologies.
- Improved communication: Working in collaborative teams fosters better communication, ultimately leading to more impactful analysis.
To facilitate efficient collaboration, organizations should create an environment that encourages knowledge-sharing through workshops, meetings, or digital platforms.
"Statistical analysis is not an island; it thrives in the sea of teamwork and collaboration."
These practices form the backbone of successful stat analytics. They facilitate thorough analyses and ultimately lead to informed decision-making in organizations. By understanding and implementing these principles, professionals can significantly improve the quality of their analytical outputs.
Finale
The conclusion of this article serves to emphasize the significance of understanding stat analytics and its multifaceted aspects. As data continues to proliferate in various sectors, mastering statistical analysis becomes vital for informed decision-making. Stat analytics provides a framework for transforming raw data into actionable insights. This transformation aids organizations in navigating complexities, enhancing efficiency, and ultimately securing a competitive advantage.
Summary of Key Points
- Stat analytics integrates statistical methods for data analysis, fostering a structured approach to extracting insights.
- Applications span multiple industries, including business intelligence, healthcare, finance, and marketing.
- Key methodologies like regression analysis and hypothesis testing enhance analysis accuracy, guiding strategic decisions.
- Ethical considerations such as data privacy and bias are critical in conducting responsible analytics.
- Future trends indicate a growing reliance on AI and real-time data processing, reshaping the landscape of stat analytics.
The importance of these elements cannot be understated. By harnessing the full spectrum of capabilities offered by statistical methods, professionals can better interpret data and implement evidence-based strategies.
Looking Ahead
As we look forward, the landscape of stat analytics will continue to evolve. New technologies and methodologies will emerge, offering fresh opportunities for analysis and insight generation. Continuous education in statistical principles and new tools will be essential for professionals seeking to maintain relevance in this dynamic field.
Moreover, the integration of artificial intelligence presents intriguing possibilities for enhancing predictive analytics. Organizations that adapt to these changes will likely experience enhanced operational efficiency and improved decision-making processes.