Self-Service Data Preparation: Enabling User Autonomy
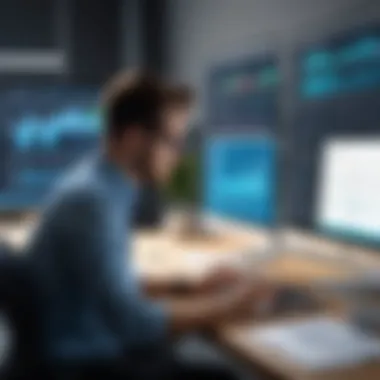
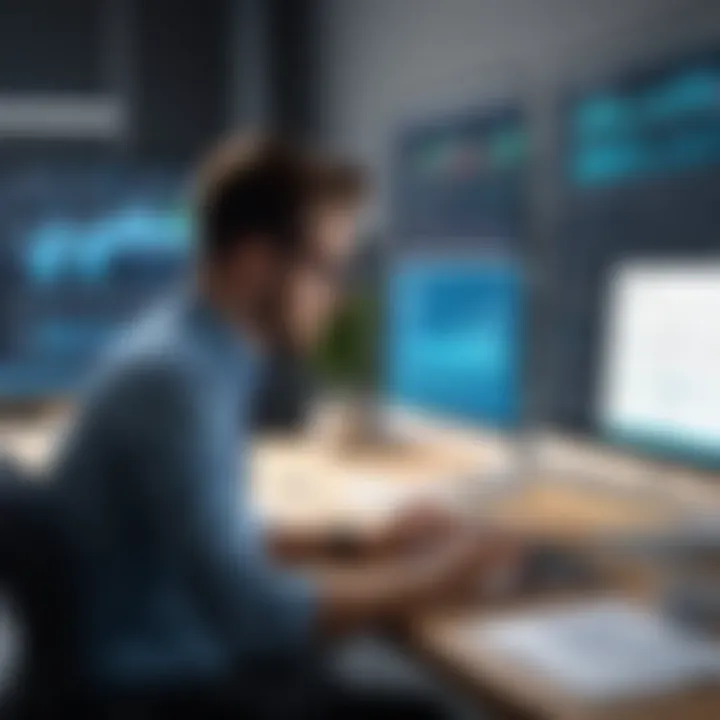
Intro
In an era where data drives decisions, the ability for users to manage their own data effectively is essential. Self-service data preparation allows users to perform data management tasks independently. This autonomy minimizes reliance on IT departments and promotes efficiency across organizations. By simplifying processes, self-service tools enable users to extract significant insights from their data without deep technical expertise.
This article delves into the methodologies, tools, and best practices that are crucial for fostering self-service data preparation. It will examine the implications for modern organizations, discussing both opportunities and challenges in implementation. Key areas of focus include assessing software needs, understanding market trends, and defining performance metrics to gauge the effectiveness of these self-service solutions.
Software Needs Assessment
Identifying User Requirements
Understanding user requirements is the cornerstone of implementing successful self-service data preparation. Users come from diverse backgrounds and have varying levels of data literacy. Hence, gathering inputs on their specific needs is paramount. Surveys and interviews can help define characteristics like:
- User Skill Levels: Assess the technical expertise of users to tailor tools accordingly.
- Data Sources Utilization: Identify which data sources users frequently access and how they interact with them.
- Desired Outcomes: Determine what insights users wish to derive from the data and what decisions need support.
A thorough identification process aids in selecting appropriate tools and ensuring that the resources provided genuinely serve user needs.
Evaluating Current Software Solutions
In the context of self-service data preparation, evaluating existing software solutions is equally important. Organizations should analyze their current tools based on several criteria, such as:
- Ease of Use: Ensuring that software is intuitive can enhance user adoption.
- Integration Capabilities: The ability to incorporate various data sources is key for any self-service tool.
- Support and Training: Availability of support resources impacts user satisfaction and success.
Consider documenting the evaluation criteria and outcomes. A comparative analysis helps identify gaps in current offerings and directs future investments in software.
Data-Driven Insights
Market Trends Overview
The landscape of self-service data preparation tools is evolving. Market research indicates a growing emphasis on user-centric design. Organizations now prioritize solutions that allow users to perform analysis with minimal assistance. The integration of machine learning and artificial intelligence into these platforms has also become prevalent. This trend provides users with more sophisticated data manipulation options without requiring extensive technical skills.
Performance Metrics
To assess the success of self-service data preparation initiatives, it is vital to establish clear performance metrics. Key performance indicators (KPIs) may include:
- User Adoption Rates: Monitor how widely the self-service tools are utilized across the organization.
- Time to Insight: Measure the speed at which users can derive meaningful insights from data, reflecting efficiency improvements.
- Quality of Insights: Evaluate the relevance and accuracy of the decisions made based on the data extracted.
Establishing these metrics not only allows organizations to gauge the effectiveness of their self-service tools but also provides a feedback loop for continuous improvement.
Self-service data preparation empowers users and promotes a culture of data-driven decision making.
By focusing on user needs and market trends, organizations can implement robust self-service data solutions. Such initiatives aid users in navigating the data landscape effectively, supporting informed decision-making and fostering productivity.
Understanding Self-Service Data Preparation
Self-service data preparation is a growing focus in the data management field. Understanding how this process works is essential for any organization aiming to maximize its data assets. Self-service enables users to handle data preparation tasks without heavy reliance on IT departments. This accessibility leads to quicker insights, allowing companies to respond faster to market needs and internal demands.
Definition of Self-Service Data Preparation
Self-service data preparation refers to tools and methodologies that empower end-users to prepare, clean, and analyze data on their own. It eliminates the bottleneck often caused by waiting for IT staff to process data requests. With certain software and applications, users can import data sets, transform data formats, and apply analytical functions as needed. This process democratizes data usage, allowing users from various departments to leverage information without intermediaries.
Historical Context
The concept of self-service data preparation emerged with advancements in technology. Initially, data preparation was primarily an IT responsibility, often involving significant time and resources. As organizations recognized the need for agility, they sought ways to decentralize data handling. Tools like Tableau and Alteryx began to popularize self-service options, changing how businesses approach data management. Over time, the evolution of cloud computing has further fueled this trend, making it easier for users to access and manipulate data. Understanding this historical development provides insight into the current capabilities and the future direction of self-service data preparation.
The Importance of Data Preparation
Data preparation plays a critical role in the landscape of data-driven decision-making. In organizations, the ability to analyze data effectively often hinges on the quality of that data. Data preparation is the process of cleaning, transforming, and organizing data to make it ready for analysis. Without proper data preparation, analysts can face substantial challenges, such as inaccurate insights and flawed conclusions, that may lead to poor business decisions.
Role in Data Analysis
Data analysis relies heavily on well-prepared datasets. When data is organized correctly, analysts can draw insights with greater confidence. Key aspects of this role include the following:
- Accuracy: Well-prepared data ensures that insights are based on valid and reliable information. Analysts spend less time correcting errors and more time interpreting findings.
- Efficiency: Streamlining data preparation saves time and resources. Analysts can quickly access the necessary data sets, facilitating faster and more insightful analysis.
- Comprehensibility: Data that is properly formatted and categorized is easier to understand. This aids in communicating results more effectively to stakeholders.
In a typical workflow, the interaction between data preparation and analysis becomes apparent. Once data is gathered, it must first undergo cleaning processes—such as removing duplicates and addressing missing values. These actions are foundational in ensuring that subsequent analyses yield trustworthy results. As data-driven decisions become increasingly vital for success, the importance of thorough data preparation cannot be overstated.
Impact on Business Intelligence
The impact of data preparation on business intelligence (BI) is profound. BI encompasses the technologies and strategies that convert raw data into meaningful information for decision-makers. Key influences of data preparation in this area include:
- Enhanced Reporting: Accurate data leads to better reporting. Businesses can generate actionable reports that highlight trends, anomalies, and opportunities.
- Improved Decision-Making: Decision-makers benefit from the availability of high-quality insights, which are derived from well-structured data. This can significantly impact strategic planning and operational efficiency.
- Scalability: As organizations grow, their data needs evolve. Self-service data preparation tools empower users to handle increasing volumes of data effectively, making it possible to scale BI efforts.
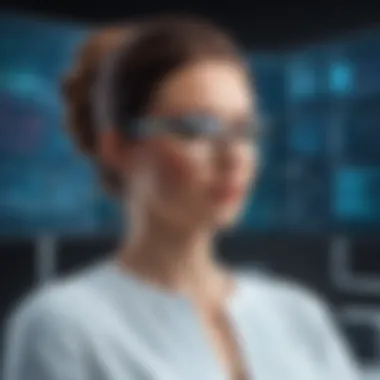
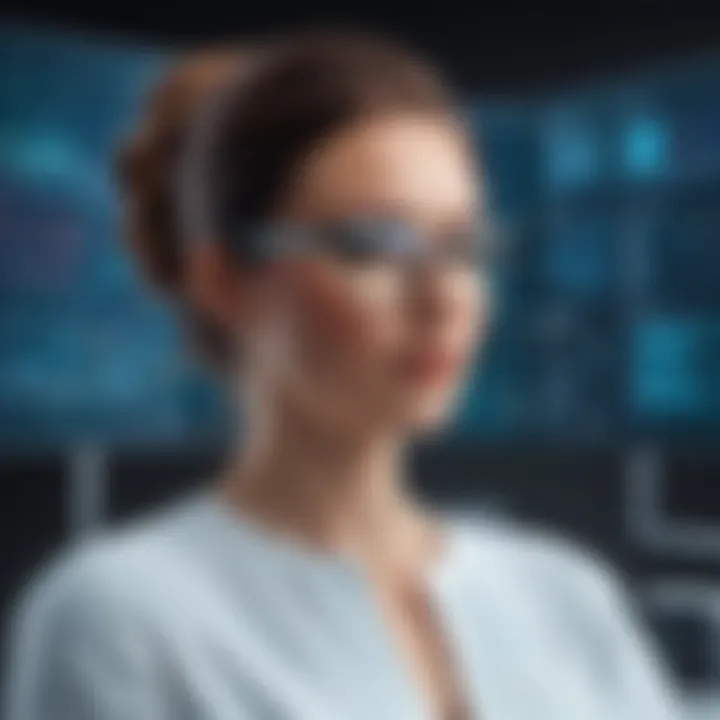
"Quality data preparation is integral to effective business intelligence."
Key Components of Self-Service Data Preparation
Self-service data preparation encompasses several essential components that facilitate user engagement and efficiency. Each component contributes to the overall goal of empowering the users by making data accessible and manageable without heavy reliance on IT. Understanding these components is crucial for organizations aiming to implement self-service data preparation successfully.
User-Friendly Interfaces
One of the most significant aspects of self-service data preparation is the user interface. A user-friendly interface is paramount to ensure that individuals with varying levels of technical expertise can work with data effectively. Intuitive designs simplify the process of importing, cleaning, and analyzing data. Users should not have to navigate through complex systems to perform tasks. Instead, streamlined workflows and clear navigation paths allow users to focus on deriving insights rather than getting lost in the mechanics of the service.
Users benefit from features such as drag-and-drop functionalities, which make handling datasets much simpler. Moreover, visual tools help users understand their data more effectively, often presenting statistics and catalogs in a more digestible format. Customization options also play a significant role, letting users create displays that match their specific needs. By prioritizing user experience, organizations can promote greater adoption of self-service tools across teams.
Automation Tools
Automation tools are critical in self-service data preparation, as they significantly enhance efficiency and accuracy. Users can set up processes that automatically clean and transform data based on pre-defined rules. This reduces manual workloads, allows users to allocate time to more strategic tasks, and decreases the likelihood of human error during data handling.
Tools for automation often include task schedulers, reminders, and integration capabilities with existing systems. For instance, integrating with popular platforms like Microsoft Excel or Google Sheets enables automatic updates of datasets within those applications. This integration minimizes disruptions in workflow and promotes seamless data access.
Additionally, automation can assist in monitoring data quality by ensuring that only clean and compliant data is being used. When users can validate their data efficiently, they can trust that the insights they derive are based on accurate foundations.
Data Quality Management
Data quality management is another crucial component in self-service data preparation. Ensuring high data quality is vital because the insights, decisions, and actions taken based on this data depend on its accuracy and relevance. Organizations must have robust mechanisms in place for validating and cleansing data to maintain its integrity.
Effective data quality management involves several practices:
- Standardizing data formats to avoid discrepancies and ensure consistency.
- Establishing validation checks that automatically flag anomalies or outliers in datasets, thereby prompting users to address potential issues promptly.
- Implementing data lineage tracking, which allows users to understand the context and history of the data they are working with.
Furthermore, training and awareness initiatives can help users understand the significance of data quality. Fostering a culture where each team member recognizes their role in maintaining data integrity can lead to long-term benefits.
With these components—user-friendly interfaces, automation tools, and effective data quality management—organizations can create a successful environment for self-service data preparation. Each aspect contributes to enhancing user autonomy while ensuring that the data used is reliable and insightful.
Technological Landscape
The technological landscape surrounding self-service data preparation is both expansive and critical to the successful adoption of these methodologies. Understanding this landscape enhances the ability of users to navigate their data environments effectively. It encompasses various software solutions, deployment options, and the evolving needs of businesses wishing to leverage data with agility.
The role of technology in self-service data preparation is paramount. It provides the necessary tools for users to interactively engage with their data, streamlining processes that were previously controlled by IT professionals. This democratization of data access can significantly boost productivity and drive better decision-making.
Software Solutions
When discussing software solutions for self-service data preparation, several key players come to mind. Tools like Alteryx, Tableau Prep, and Microsoft Power BI have gained recognition for their user-friendly interfaces and comprehensive features that empower users. Each of these software options offers functionality that caters to diverse user needs, from data cleaning to visual analytics.
- Alteryx excels in data blending and transformational capabilities. Users can connect disparate data sources, perform complex calculations, and prepare data for analysis all in one platform.
- Tableau Prep provides a visual and intuitive way to clean and shape data. Its drag-and-drop feature allows users to build data flows and visualize their preparation steps in real time.
- Microsoft Power BI integrates seamlessly with existing Microsoft ecosystems, providing not only data preparation tools but also robust analytics and visualization capabilities.
These tools enhance user experience by offering functionalities that include automation of data preparation tasks, visual integrations, and methodologies that cater to varying skill sets within an organization. Choosing the right software solution also requires an understanding of specific organizational requirements and user proficiency.
Cloud vs. On-Premise Solutions
The decision between cloud and on-premise solutions greatly impacts the self-service data preparation landscape. Each has its own benefits and drawbacks, influencing how data is accessed and processed.
- Cloud Solutions:
- On-Premise Solutions:
- Accessibility is a significant advantage. Users can access data from anywhere, facilitating remote work and collaboration.
- Scalability is often easier; organizations can adapt their plans as data needs grow without significant infrastructure investment.
- However, organizations may have concerns about data security and compliance as sensitive information is stored off-site.
- These systems offer enhanced control over data security. Companies can manage sensitive information in a manner that meets their unique compliance requirements.
- Performance can often be higher with local systems, especially for large datasets, since they are not restricted by internet bandwidth.
- The downside is that the initial setup costs can be higher, and maintenance becomes the organization's responsibility, requiring ongoing technical support.
Choosing the right deployment option should be informed by organizational goals, data sensitivity, regulatory requirements, and user accessibility needs. Evaluating these factors ensures that companies can successfully implement self-service data preparation methodologies.
User Empowerment
User empowerment is a foundational element in the discussion of self-service data preparation. It encapsulates the notion of enabling users to navigate data independently, without always relying on IT departments. This approach can significantly enhance productivity and foster a culture of data-driven decision-making across an organization.
By reducing the barriers to entry, self-service data preparation tools enable users from various backgrounds to engage with data. This accessibility brings several benefits, including increased efficiency, faster decision-making, and greater satisfaction among users. Furthermore, when users feel empowered to analyze their data, they are likely to derive deeper insights that can lead to more informed business strategies.
Reducing IT Dependency
Reducing dependency on IT is central to the idea of self-service data preparation. Traditionally, when employees needed access to data or required specific analysis, they would reach out to IT teams. This process could be slow and cumbersome, often leading to frustration and bottlenecks in decision-making.
With self-service tools, users can take control of their data journey. They can access data themselves, perform analyses, and generate reports without waiting for IT’s involvement. This shift not only frees up the IT staff to focus on more complex tasks but also promotes autonomy among users.
Consider the following:
- Speed: Users can quickly create their reports and insights, drastically reducing the time spent waiting for IT to respond.
- Flexibility: Users can explore data on their own terms, allowing for more creative solutions and strategies.
- Ownership: By managing their data, users develop a sense of ownership, fostering initiative and responsibility in handling data-related tasks.
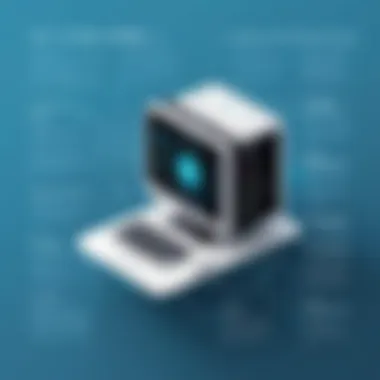
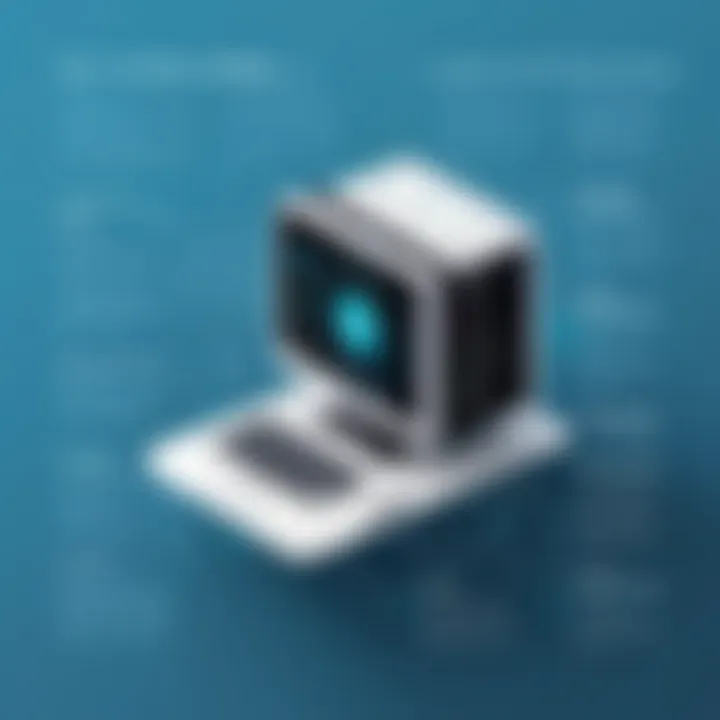
Fostering Data Literacy
Fostering data literacy is critical in the movement toward user empowerment. As organizations adopt self-service data preparation, employees need to possess a fundamental understanding of data principles. This knowledge enables them to effectively use available tools and extract meaningful insights.
Promoting data literacy involves several key areas:
- Training Programs: Tailored training sessions can educate employees on how to utilize self-service tools. This guidance ensures they are comfortable navigating their data.
- Resources and Documentation: Providing written materials, tutorials, and user guides can help users develop competence in data analysis.
- Mentorship and Peer Support: Encourage experienced users to support their colleagues, fostering a collaborative environment where data skills can flourish.
Empowering users through data literacy not only enriches their ability to work with data but also encourages a broader culture of analytical thinking within the organization. The more skilled users become, the more they can contribute to strategic business initiatives effectively.
"Empowering users equips them with the skills necessary to navigate the complexities of data and leverage it for strategic advantage."
In summary, user empowerment goes beyond mere access to tools. It embodies a holistic approach where users understand the value of data and have the capability to manipulate it effectively for their needs. This transformation is essential for fostering a data-driven culture in organizations.
Challenges in Self-Service Data Preparation
Self-service data preparation holds promise for enhancing user engagement with data. However, it also brings several challenges that organizations must address. Understanding these challenges can facilitate proper strategies for navigating them. The focus on self-service access to data does not eliminate the complexities involved. Rather, these complexities evolve, requiring a comprehensive response.
Data Governance Issues
Data governance is a critical component in self-service data preparation. It encompasses the management of data availability, usability, and security. When users have more control over data, the risk of data mismanagement increases. Often, individuals may not be fully aware of data lineage or the implications of data alteration.
Proper governance frameworks need to be established to protect the integrity of data. This includes:
- Defining clear roles and responsibilities for data stewardship.
- Implementing policies that guide how data can be accessed, transformed, and shared.
- Utilizing metadata management to ensure users understand the context of the data.
An effective strategy must balance ease of access with adherence to compliance regulations. Without this balance, organizations could face data inconsistencies and adverse legal consequences.
Security Concerns
The rise of self-service data preparation has transformed not only how data is accessed but also how securely it must be treated. Users in a self-service environment require certain permissions to handle sensitive information. The unauthorized access to data can lead to significant breaches, making security protocols a paramount concern.
Organizations should consider the following:
- Implement role-based access controls that limit data exposure based on user roles.
- Employ data masking techniques to protect sensitive information when shared.
- Regularly conduct security audits to identify vulnerabilities.
Maintaining a robust security posture reduces risks while promoting the benefits of user empowerment. This is essential in establishing trust between technology use and data management practices.
Data governance and security are foundational to successful self-service data preparation. Without addressing these challenges, organizations risk encountering operational inefficiencies and compliance failures.
Successful navigation of these issues ensures that self-service users can engage with data confidently while minimizing potential risks.
Best Practices for Implementation
Implementing self-service data preparation effectively is a multifaceted endeavor. It involves not only the adoption of technology but also the cultivation of an organizational culture that supports data empowerment. The importance of best practices in this area cannot be overstated. They not only smooth the transition to self-service but also ensure sustainability and scalability. Key elements include:
- Alignment with Business Goals: Ensure that self-service initiatives align with overall business objectives. This creates relevance and secures buy-in from stakeholders.
- User-Centric Design: Focus on developing tools that prioritize user experience. A design that is intuitive will promote adoption and reduce the learning curve.
- Regular Feedback Mechanisms: Establish a process for collecting and implementing user feedback. This promotes continuous improvement and addresses pain points early on.
By attending to these specifics, organizations can create a strong foundation for self-service data preparation.
Creating a Clear Strategy
The first step in efficient implementation is creating a clear strategy. A well-defined strategy guides the organization in establishing self-service data preparation processes. It should encompass several considerations:
- Assess Existing Capabilities: Understand current data management processes and systems. Identify gaps and opportunities for enhancing self-service capabilities.
- Define Objectives: What does the organization hope to achieve? Clarity around objectives such as reducing time spent on data preparation or increasing data accessibility will help focus efforts.
- Document Processes: Create thorough documentation for users to refer to. This should include step-by-step guides on how to access and prepare data for analysis.
These measures ensure that self-service implementations are intentional and directed. Without a clear strategy, efforts may become disjointed and less effective, undermining the potential benefits of self-service data preparation.
Training and Support
The success of self-service data preparation is heavily reliant on robust training and support systems. Users must feel equipped and confident to use the new tools available to them. Training initiatives should cover:
- Onboarding Sessions: Conduct comprehensive onboarding sessions for new tools. These sessions can help familiarize users with functionalities and promote competency.
- Ongoing Learning Resources: Provide continually updated learning materials, such as user guides and how-to videos. This allows users to refresh their knowledge as needed.
- Establish Support Channels: Ensure that users have access to help when they encounter difficulties. This can range from a dedicated helpdesk to community forums where users can seek advice from peers.
Incorporating training and support as vital components of implementation will foster a culture of continuous improvement. Users who receive adequate training and support are more likely to embrace self-service and leverage its full potential.
Case Studies
Case studies serve as an essential component in understanding the practical implications of self-service data preparation. They provide real-world examples that illustrate how various organizations implement this approach. Through these implementations, businesses can gain insights into effective strategies, challenges faced, and the resulting benefits. By analyzing these case studies, one can appreciate the transformative power of self-service data preparation.
- Examples of Diverse Applications: Different organizations across industries such as healthcare, finance, and retail have embraced self-service data preparation. Each case study reflects the unique needs and challenges of the sector. For instance, a major health organization might focus on patient data management, while a retail company may prioritize customer journey analysis.
- Benefits Realized: These implementations often enhance efficiency and make data insights more accessible. Organizations have reported quicker decision-making processes and improved data quality. Such benefits underscore the value of empowering users at all levels.
- Critical Considerations: While examining these case studies, it is important to note that challenges persist. Issues related to data governance and user training often emerge. Recognizing these factors allows organizations to prepare adequately and mitigate potential risks.
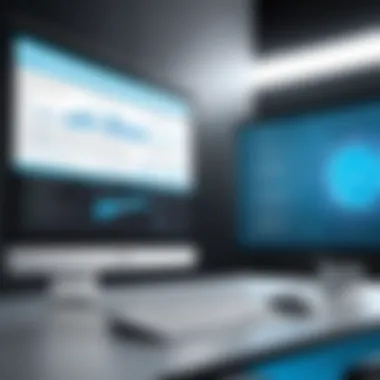
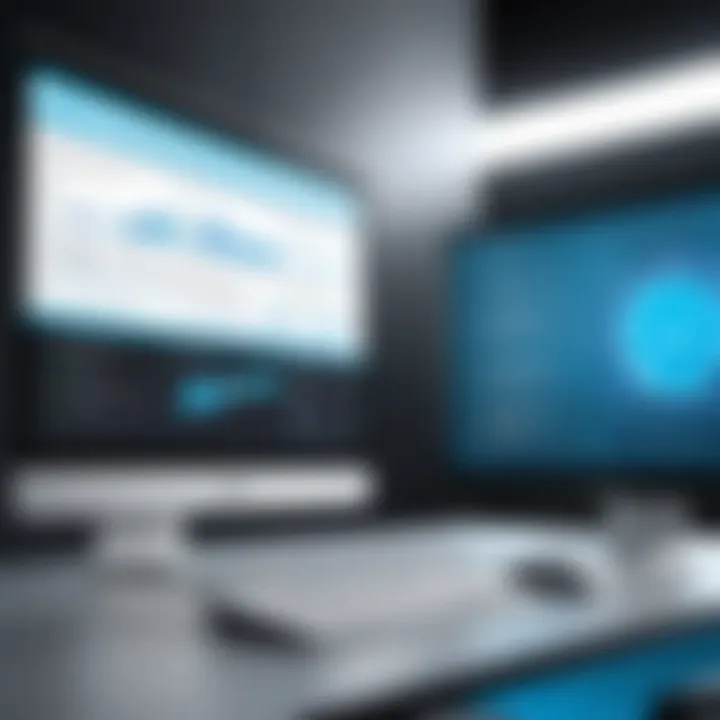
Successful Implementations
Successful implementations of self-service data preparation reveal practical strategies. A case that stands out involves a multinational tech company. They aimed to reduce reliance on their IT department for data analytics and reporting.
They introduced an intuitive platform, enabling employees to create reports without extensive technical skills. The results were impressive. Within six months, productivity increased by 30%. Business units became adept at using data for initiatives like product development based on customer feedback.
Key elements that contributed to this success included:
- Comprehensive Training: The organization prioritized training sessions to boost user confidence.
- User-Centric Design: The platform was designed with user experience in mind, ensuring it was intuitive.
- Ongoing Support: They offered ongoing support through FAQ sessions and help desks.
This case illustrates that, with the right tools and support, organizations can significantly enhance their data capabilities.
Lessons Learned
Lessons gleaned from case studies on self-service data preparation highlight essential attributes that should be considered.
A prominent lesson involves maintaining a balance between user autonomy and governance. One financial services firm learned this after encountering data breaches. While encouraging user independence, they discovered that a lack of oversight led to unauthorized data access. Consequently, they strengthened their data governance policies while still fostering a self-service culture.
Key lessons include:
- Implement Strong Governance Policies: This is crucial to protect sensitive information while allowing users to operate independently.
- Adapt Training Programs: Regular updates to training programs can ensure users are aware of evolving platform features and best practices.
- Gather Feedback and Iterate: Engaging users in feedback loops can lead to continuous platform improvements and satisfaction.
Ultimately, these lessons offer a roadmap for organizations. They reflect not only the successes but also the pitfalls to avoid, ensuring that users fully realize the potential of self-service data preparation.
Future Trends in Self-Service Data Preparation
Future trends in self-service data preparation denote transformative shifts that will define how organizations approach their data management strategies. Understanding these trends is critical for businesses aiming to maintain a competitive edge in increasingly data-centric markets. As technology continually evolves, the integration of advanced tools empowers users to harness data dynamically and efficiently. This section will explore how artificial intelligence and machine learning, as well as the integration with advanced analytics, are becoming pivotal in shaping self-service data preparation.
Artificial Intelligence and Machine Learning
Artificial intelligence and machine learning are breaking barriers in data preparation. These technologies automate learning from data, resulting in smarter and faster data handling. By streamlining the preparation phase, AI enhances user autonomy, allowing individuals with limited technical knowledge to analyze data effectively.
Key benefits include:
- Increased Efficiency: AI tools can process vast datasets quickly, enabling real-time analysis without human intervention.
- Enhanced Accuracy: Machine learning algorithms improve over time, reducing errors in data preparation and analysis.
- Predictive Insights: AI can forecast trends based on historical data, offering users predictive capabilities that inform decision-making.
However, it is essential to consider factors like:
- Data Bias: Machine learning models can perpetuate biases found in training data. Ensuring diverse datasets is crucial.
- Skills Gap: Organizations might need to invest in training to ensure users can leverage AI tools effectively.
Adopting AI and ML will significantly empower users, creating more accessible and efficient ways to interact with data.
Integration with Advanced Analytics
Integrating self-service data preparation with advanced analytics tools is becoming a norm. This convergence provides users with comprehensive insights derived from prepared data, enabling more informed decisions. Advanced analytics encompass statistical analysis, data mining, and predictive modeling, pushing the boundaries of what users can achieve independently.
Benefits of integration include:
- Holistic Understanding: Users derive comprehensive insights by merging data preparation with analytics, providing a complete picture of data trends.
- Dynamic Reporting: Advanced analytics enable users to create dynamic dashboards that visualize their findings instantly.
- Collaboration Facilitated: Integration leads to enhanced collaboration across departments as data is prepared and analyzed using unified tools.
Nevertheless, organizations must remain aware of:
- Tool Complexity: A balance must be struck between functionality and usability. Overly complex tools can intimidate users rather than empower them.
- Interoperability: Ensuring that different tools work seamlessly together can pose technical challenges that require careful planning.
The integration of advanced analytics is essential for organizations to fully leverage their prepared data, enabling users to extract actionable insights without reliance on IT.
In summary, the future of self-service data preparation will see a prominent role for AI, machine learning, and advanced analytics. These technologies not only enhance user empowerment but also pave the way for more innovative and effective data management practices.
Culmination
The conclusion of this article emphasizes the significance of self-service data preparation in contemporary data environments. As organizations increasingly rely on data for strategic decision-making, the accessibility of data preparation tools empowers users, fostering a culture of data-driven insights. Key elements include simplifying processes and enhancing user autonomy. This method encourages users to engage directly with data, shifting reliance away from IT departments.
Summarizing Key Points
In summary, self-service data preparation brings several critical advantages:
- Empowerment of Users: Users can manipulate and analyze data without extensive IT intervention, increasing productivity and flexibility.
- Efficiency: Reducing the bottleneck created by IT allows for quicker insights and timely decision-making.
- Advanced Tools: A range of software solutions exists, streamlining various data preparation tasks.
- Data Literacy: Training users on these tools not only supports their empowerment but enhances overall data literacy within the organization.
The challenges faced, such as data governance and security concerns, are essential to recognize. Balancing these risks with the benefits of self-service capabilities leads to a productive approach.
The Path Forward
Looking ahead, the trajectory of self-service data preparation will be shaped by technology and organizational practices. Investment in tools that integrate artificial intelligence and machine learning will enhance user capabilities. Furthermore, organizations must prioritize training to ensure users can effectively utilize these tools. An emphasis on data governance will also become increasingly relevant, guiding users on ethical data handling.
Engaging with these trends will allow businesses to maintain competitiveness and harness the full potential of their data assets. The focus should remain on continuous improvement in processes, tools, and user capabilities, ensuring that self-service data preparation becomes a fully integrated aspect of organizational strategy.
"The future of work will involve users not merely consuming data but actively shaping it."
By embracing self-service data preparation, organizations can transform data into actionable insights, ultimately leading to better outcomes and a more informed workforce.