Comprehensive Insights on Discovery Data Pricing
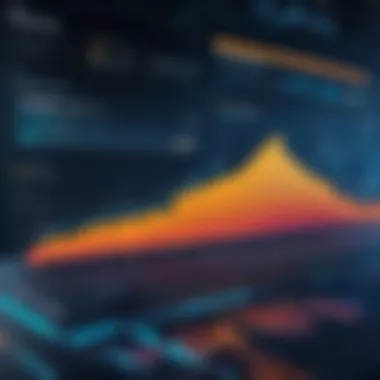
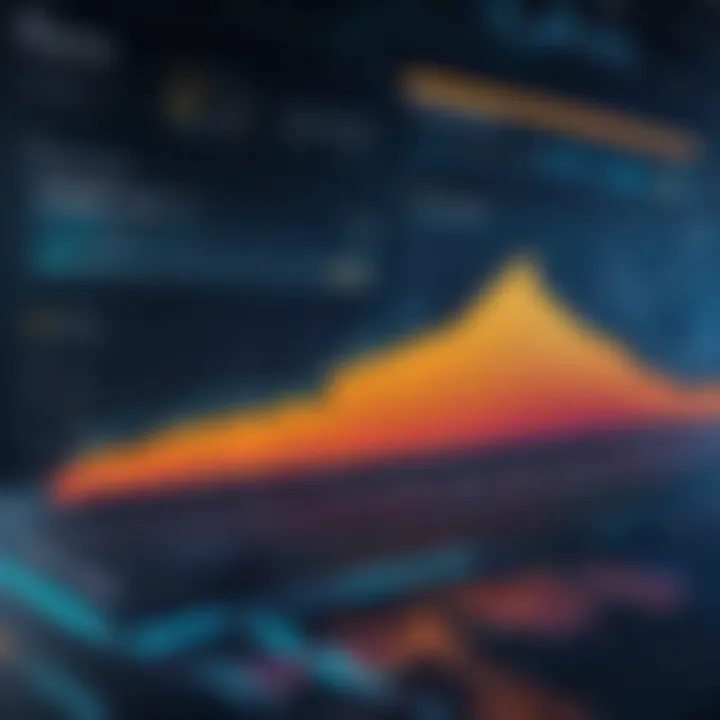
Intro
The pricing of discovery data plays a critical role in shaping how organizations manage their information resources. Navigating this landscape requires a clear understanding of the variables at play. The complexity of pricing models can lead to confusion, making it essential for decision-makers to differentiate between factors that matter most. This section aims to lay the groundwork for a thorough comprehension of these components and their implications for businesses.
Software Needs Assessment
Assessing software needs involves more than recognizing the tools available on the market. It is about aligning those tools with organizational goals and user requirements. Understanding these needs sets the foundation for effective data discovery solutions.
Identifying User Requirements
Effective data discovery software must cater to the specific requirements of its users. This means engaging with stakeholders to extract their pain points and needs. Companies should consider factors such as:
- Data volume: Understanding how much data will be processed.
- User expertise: Differentiating between technical and non-technical users.
- Integration capabilities: Ensuring compatibility with existing systems.
- Security needs: Assessing the level of protection required for sensitive information.
A clear set of user requirements can foster the appropriate focus on features that support an organization’s data strategy.
Evaluating Current Software Solutions
Once user needs are established, organizations can analyze current software solutions that address these criteria. This evaluation process should focus on:
- Functionality: Does the software meet the defined requirements?
- Cost structures: Investigating different pricing models available.
- Vendor reputation: Researching the track record of software providers.
Comparing solutions through demos or trials might provide additional insights into their effectiveness in real-world scenarios.
Data-Driven Insights
Data-driven insights are fundamental for making informed decisions in a rapidly evolving market. Staying up-to-date with available trends ensures that businesses can navigate the complexities of pricing frameworks thoughtfully.
Market Trends Overview
The landscape of discovery data pricing is influenced by various market trends. Organizations must recognize the impacts of:
- Technological advancements: New features that can alter pricing structures.
- Shifts in consumer demand: Trends affecting the level of customization needed.
- Ecosystem changes: The rise of integrated platforms may shift pricing strategies.
Staying informed about these trends can aid stakeholders in predicting future pricing shifts and optimizing their investments.
Performance Metrics
Performance metrics serve as a useful tool for assessing the effectiveness of data discovery software. Companies should consider metrics such as:
- Cost per data transaction: Understanding economic efficiency.
- User satisfaction ratings: Gathering feedback directly from users.
- System scalability: Evaluating the software’s ability to grow with business needs.
Utilizing performance metrics allows for data-backed decisions, enhancing the overall evaluation of cost versus benefit.
"Understanding pricing structures in discovery data is not just about numbers, it is about strategic alignment with business goals."
By comprehending these foundational aspects, decision-makers can approach discovery data pricing with a clearer perspective, leading to better outcomes for their organizations.
Foreword to Discovery Data Pricing
Understanding discovery data pricing is crucial in today's data-driven world. As enterprises increasingly rely on diverse data sources, the ability to access, analyze, and interpret this data is becoming paramount. Discovery data refers to the relevant information that organizations discover during their data management processes. This concept encompasses various data types and formats, often leading to significant implications concerning costs.
Definition of Discovery Data
Discovery data can be defined as the information obtained through data exploration techniques. This process involves sifting through extensive data sets to uncover patterns, trends, and anomalies. The types of discovery data can vary widely, including structured data from databases, unstructured data from documents and emails, and semi-structured data such as logs or JSON files.
The relevance of discovery data is underscored by its potential to unlock insights that inform strategic decision-making. Businesses can leverage this information to optimize operations, enhance user experiences, and drive innovation. Improper handling or mispricing of discovery data can lead to inefficiencies and financial discrepancies.
Importance of Discovery Data in Modern Enterprises
The importance of discovery data in modern enterprises cannot be overstated. As organizations collect enormous volumes of data, the challenge lies not only in storage but also in effective utilization. Effective discovery data practices enable companies to:
- Improve Decision-Making: Access to comprehensive data insights ensures that decisions are based on factual evidence rather than intuition.
- Enhance Compliance: Proper discovery helps organizations meet regulatory requirements by ensuring data integrity and traceability.
- Drive Innovation: Analyzing discovery data can reveal trends that guide research and development initiatives, ultimately leading to new product offerings.
- Increase Efficiency: Streamlined data discovery processes reduce time spent on data retrieval and allow teams to focus on analysis rather than search efforts.
In summary, discovery data pricing is a foundational aspect of modern business operations. As enterprises continue to evolve and adapt, understanding the nuances of this pricing landscape is essential. It enables organizations to make informed choices about tools, implementations, and ongoing management of their data, positioning them for long-term success.
Understanding Pricing Models
Understanding pricing models is essential in navigating the intricate domain of discovery data pricing. Pricing models directly influence the budget allocation for data tools. This section will clarify various models used in the industry. The models can affect cost predictability, budgeting, and general financial planning within an organization. It is crucial for tech-savvy individuals, business professionals, and IT specialists to comprehend the options available. This knowledge facilitates well-informed decisions when selecting the appropriate discovery data tool.
Fixed Pricing Structures
Fixed pricing structures provide a predictable cost model. In this arrangement, clients pay a flat fee for access to specific features or capabilities. This model can be advantageous for budgeting purposes. Companies can forecast expenses without unexpected costs.
Benefits of fixed pricing include:
- Budget Certainty: Companies can commit to plans knowing the maximum costs.
- Simplicity: Understanding what is included in a package is straightforward.
- Stability: No sudden price changes occur over time.
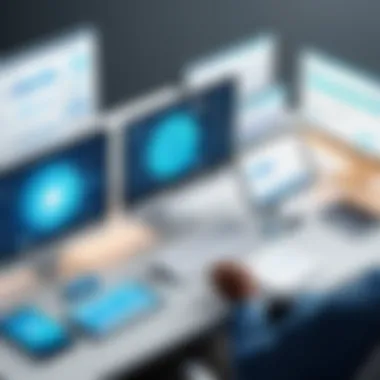
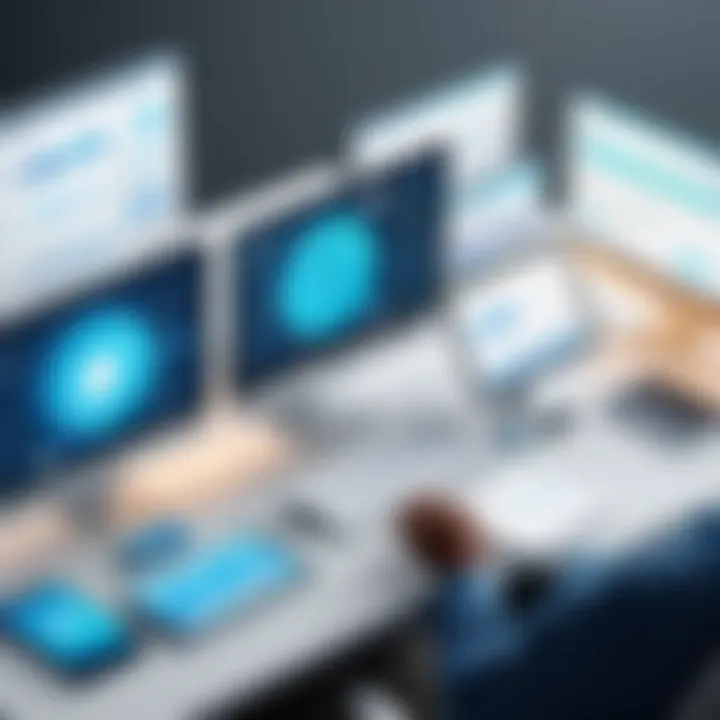
However, fixed pricing can also have restrictions. Sometimes, features that a user needs may be locked behind higher-tier pricing plans. Companies must assess their expected usage patterns carefully before settling on a fixed plan.
Variable Pricing Models
In contrast to fixed pricing, variable pricing models adjust costs based on usage or the volume of data. This flexibility can suit companies whose data needs fluctuate. Organizations may find that they only pay for what they actually use.
Considerations for this model include:
- Cost-Efficiency: Potentially lower costs if usage is low.
- Scalability: Companies can scale their spending as their data needs grow.
- Complexity: It may be difficult to predict future costs based on variable usage.
Organizations should evaluate the long-term implications of choosing a variable pricing model. They may end up spending more if their data processing needs increase significantly over time.
Per-User vs. Per-Feature Pricing
Per-user and per-feature pricing models are distinct approaches. In the per-user model, costs are determined by the number of users accessing the tool. This arrangement may work well for companies with many users who require access to the same features. It can lead to significant expenses if the user base grows unexpectedly.
On the other hand, per-feature pricing charges based on the specific functionalities chosen. Companies might pay only for the features they need without additional costs for unused options. This can be beneficial for organizations that require distinct capabilities.
Benefits of each pricing model include:
- Per-User Pricing:
- Per-Feature Pricing:
- Simplifies cost calculation for teams with large user bases.
- Promotes collaboration among users.
- Offers a tailored approach based on company needs.
- Helps avoid costs associated with features not in use.
Factors Influencing Discovery Data Pricing
Understanding the factors influencing discovery data pricing is critical for companies looking to optimize their data management costs. Each element plays a significant role in determining the price point of discovery data tools. In an era where data is paramount, grasping these factors helps organizations make informed and strategic decisions regarding their data solutions.
Volume of Data Processed
One of the primary factors impacting pricing is the volume of data processed. Higher data volumes typically lead to increased costs. Vendors often set their prices based on how much data an organization generates or uses. For instance, solutions like Microsoft Azure and Amazon Web Services use tiered pricing models that charge customers based on the amount of data stored or processed.
When estimating costs, it is vital for businesses to accurately gauge their data volume. This involves not only assessing current data but also forecasting future growth. Ignoring data growth can lead to under-budgeting, resulting in unforeseen expenses.
Complexity of Data Structures
The complexity of the data structures involved also affects pricing. Simple data sets may incur lower costs, while complex ones, involving multiple formats or large datasets, typically lead to higher prices. Solutions that require advanced algorithms or extensive processing capabilities can present additional costs.
For example, a business dealing with structured data will have different pricing considerations compared to one handling unstructured data. The latter often needs specialized tools, which can drive up fees significantly. Evaluating the complexity of data structures can help businesses choose appropriate tools and understand their potential costs.
Integration with Existing Systems
Another critical consideration is the integration of discovery data tools with existing systems. Smooth integration can save time and reduce overall costs. If a tool requires extensive customization or fails to integrate well, expenses can rise sharply. Companies should evaluate how well a particular solution fits into their current setup.
For instance, tools like Tableau or Splunk are praised for their versatility and ease of integration with various data sources, potentially lowering long-term costs. Thus, businesses must assess both initial setup costs and ongoing integration expenses when evaluating pricing.
User Support and Services Offered
User support and the range of services provided can significantly influence discovery data pricing. Comprehensive support helps ensure that users can fully leverage the tool's capabilities. Solutions with dedicated customer support tend to be more expensive, but the investment can lead to increased efficiency and reduced downtime.
For example, platforms like Qlik offer extensive technical support and training programs, which can justify higher pricing depending on the needs of the company. Regular updates, customer training, and accessible support channels are valuable components of pricing that companies should consider when choosing a tool.
"When evaluating discovery data tools, companies should not merely focus on upfront costs. The ongoing support and integration are often where the real value lies."
Market Trends in Discovery Data Solutions
The landscape of discovery data solutions is constantly evolving. Understanding the market trends is crucial for decision-makers. These trends reveal how businesses adapt to changing environments and technological advancements. They also provide insights into future directions. A solid grasp of these trends allows organizations to make strategic choices about tools and systems that align with their goals.
Rising Demand for Data Analytics
In recent years, there has been a noticeable increase in the demand for data analytics. Companies are recognizing the significance of data in informing their strategies and operations. Decision-makers are prioritizing data-driven approaches to enhance performance and gain a competitive edge.
Reasons for this demand include:
- The explosion of data generated by business operations.
- The need to convert data into actionable insights.
- The growing importance of personalized customer experiences.
This demand is leading organizations to invest in advanced data analytics tools. They are looking for capabilities that allow deeper insights into customer behaviors, market opportunities, and operational efficiencies. Tools that offer predictive analytics, real-time data processing, and visualization capabilities are becoming popular choices.
Emergence of AI-Driven Tools
AI technology is getting embeded in data discovery solutions. The use of AI-driven tools is revolutionizing how organizations approach data analysis. These tools can process vast amounts of information rapidly and deliver insights that manual processes might miss.
Key benefits of AI integration include:
- Enhanced analytical capabilities through machine learning.
- Automation of routine data processing tasks.
- Ability to uncover patterns and trends not readily visible to humans.
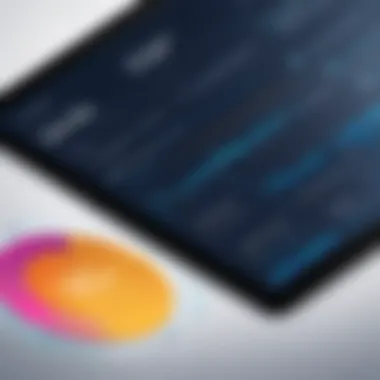
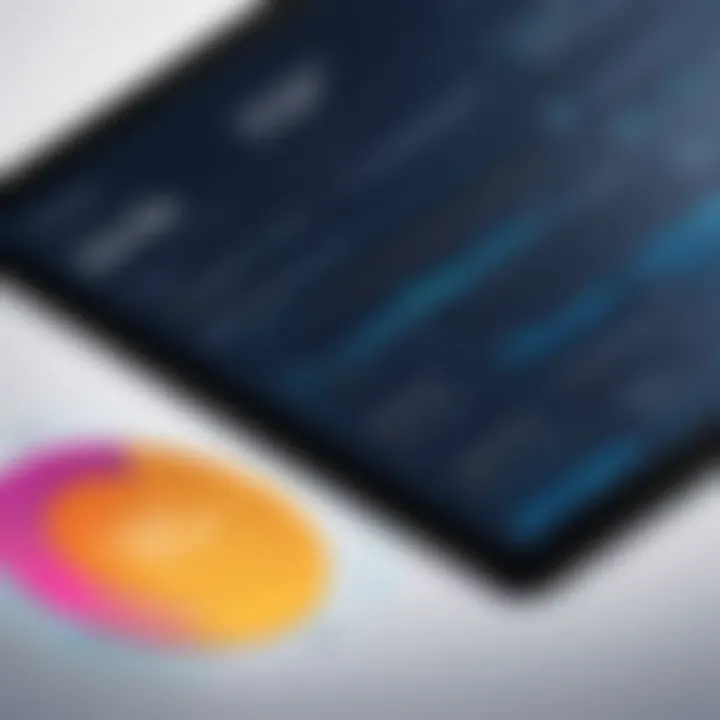
AI also improves the accuracy of insights. Companies that adopt these solutions often see faster decision-making and refined strategies. They can anticipate market changes and respond effectively.
Shift Towards SaaS Solutions
Software as a Service (SaaS) models are becoming increasingly attractive for organizations. This shift is part of a broader trend towards cloud-based solutions in tech infrastructure. SaaS offers flexibility, scalability, and accessibility, which are key considerations for modern enterprises.
Notable advantages of SaaS solutions are:
- Reduced upfront costs due to subscription-based pricing models.
- Easy access to updates and new features as suppliers manage the software.
- Enhanced collaboration through cloud connectivity among different teams.
Transitioning to SaaS can streamline workflows, improve collaboration, and often leads to cost savings. As businesses continue to embrace digital transformation, the trend toward SaaS solutions for data analytics will likely persist.
The integration of AI and SaaS in data discovery tools is not just a trend but a pivotal shift. Companies that understand and adapt to these market changes will position themselves favorably for future challenges.
Comparative Analysis of Leading Tools
Conducting a comparative analysis of leading discovery data tools is crucial for organizations looking to optimize their data management capabilities. This process helps to assess the available options in the market, allowing businesses to make informed decisions regarding which tool best meets their specific needs. Each tool offers unique features, pricing models, and user experiences, making it essential for decision-makers to evaluate these factors comprehensively.
Key Players in the Market
In the realm of discovery data tools, several key players dominate. Each of these tools has established a strong reputation due to their innovative features and robust performance.
- Tableau: Known for its powerful data visualization capabilities, it allows users to create interactive and shareable dashboards.
- Qlik: It offers associative data modeling and analytics, making it easy to explore data from multiple sources.
- Alteryx: Focused on data preparation, it provides tools for data blending and advanced analytics.
- Power BI: Microsoft's solution offers seamless integration with other Microsoft products and provides comprehensive analytics capabilities.
These tools have gained traction because of their ability to meet the diverse requirements of various businesses. Analyzing their offerings can provide insight into what might work best for a given enterprise.
Strengths and Weaknesses of Each Tool
Each of the leading tools brings distinct strengths and some weaknesses. Understanding these can shape a better purchasing decision:
Tableau
Strengths:
- Excellent visualization functionalities.
- User-friendly interface.
- Strong community support.
Weaknesses:
- Can be costly at scale.
- Limited advanced analytics features compared to some competitors.
Qlik
Strengths:
- Powerful associative engine enabling dynamic exploration.
- Robust data integration capabilities.
Weaknesses:
- Steeper learning curve for new users.
- Sometimes requires more configuration.
Alteryx
Strengths:
- Exceptional data preparation and blending capabilities.
- Support for complex analytics.
Weaknesses:
- Higher pricing for advanced features.
- May be too complex for simple tasks.
Power BI
Strengths:
- Cost-effective, especially for Microsoft users.
- Easy to implement and learn.
Weaknesses:
- Limited customization options.
- Can be slow with very large datasets.
Understanding the strengths and weaknesses of each tool can lead to improved decision-making, helping businesses align their choice with their strategic objectives. As the landscape of discovery data pricing evolves, staying informed about the capabilities of leading tools remains critical.
Cost-Benefit Analysis of Discovery Data Tools
Understanding the cost-benefit analysis of discovery data tools is crucial for organizations looking to leverage these technologies effectively. This analysis allows decision-makers to weigh the financial implications against the benefits gained by implementing these tools. The aim is to assess whether the potential return justifies the investment. Optimal resource allocation is essential in today’s competitive landscape, hence a meticulous evaluation is necessary.
Evaluating Financial Impact
Evaluating the financial impact involves assessing the total cost incurred while employing discovery data tools. Costs may include not only the purchase price but also installation, training, maintenance, and support.
Key considerations include:
- Initial Costs: Prices for software licenses or subscription fees should be fully understood.
- Ongoing Expenses: This includes subscription renewals, updates, and cloud storage costs.
- Training Costs: Ensuring staff are capable of utilizing the tool effectively is often overlooked.
- Opportunity Costs: Assessing what other projects or investments are sacrificed for this implementation is also essential.
Carefully documenting these costs provides a clearer financial picture. Companies can compare existing solutions with new options, highlighting fields in which the new tool may be more economical or effective.
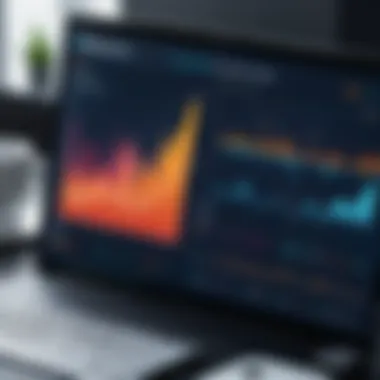
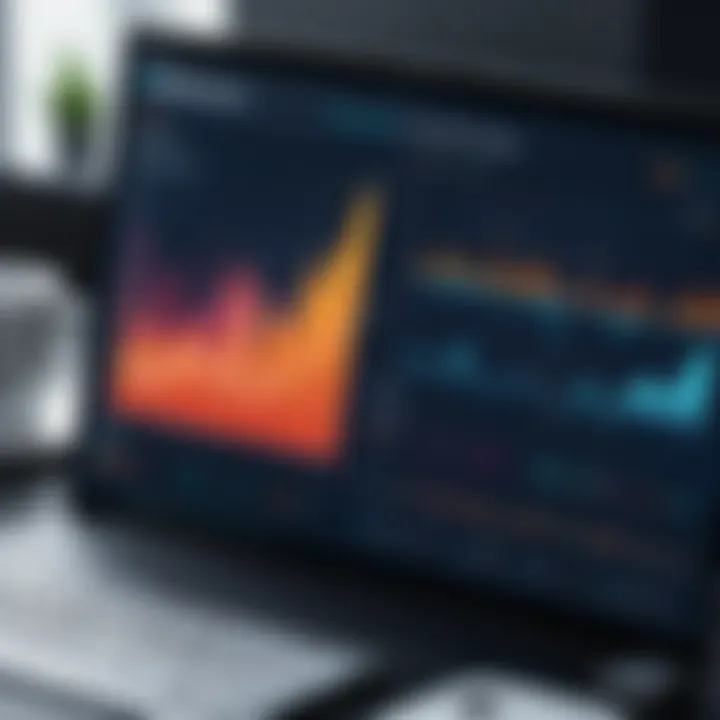
ROI Considerations
Return on Investment (ROI) calculations help determine the effectiveness of the discovery data tools in relation to the costs incurred. Calculating ROI involves measuring the benefits gained from the tool against its entire lifecycle cost.
Factors influencing ROI include:
- Increased Efficiency: How much time the tool saves employees in processing data.
- Improved Decision-Making: Analyze how better data insights lead to increased sales or improved customer satisfaction.
- Cost Savings: Investigate whether reducing errors or manual labor translates to significant savings over time.
To derive an accurate ROI, organizations must consider both qualitative and quantitative benefits.
This comprehensive analysis helps business leaders make informed decisions on whether investing in discovery data tools aligns with their strategic goals.
Ultimately, a thorough cost-benefit analysis equips organizations to make decisions that enhance productivity and provide substantial financial returns.
Best Practices for Evaluating Options
In the realm of discovery data pricing, establishing effective evaluation practices is paramount. Organizations face myriad choices, each with distinct features and costs. Best practices not only streamline this decision-making process but also ensure that the selected tools effectively align with the organization's objectives. By adopting a structured and informed approach, businesses can avoid common pitfalls and leverage data discovery tools to their full potential.
Establishing Internal Needs
The first step in evaluating discovery data options involves a thorough assessment of internal needs. Companies must identify their specific requirements, which can vary widely based on industry, data types, and size of operations.
- Data Volume: Consider how much data needs to be processed. A larger volume often necessitates more robust tools that can handle the load efficiently.
- User Requirements: Different users may have varying levels of expertise. Assess user familiarity with data tools to ensure that the chosen solution meets their capabilities.
- Integration Needs: Existing systems should be considered to avoid complications during implementation. Look for tools that easily integrate with current data infrastructure.
Taking the time to systematically define these criteria will lay the groundwork for a successful evaluation of potential data discovery tools.
Assessing Vendor Credibility
Vendor credibility is a critical factor in the decision-making process. It is essential to delve into the vendor's background, market reputation, and the experiences of current users.
- Experience and Track Record: Investigate how long the vendor has been in business and their history in providing discovery data solutions.
- Customer Reviews and Testimonials: Seeking feedback from existing clients can provide insights into the vendor's reliability and support. This real-world perspective often reveals aspects not found in product literature.
- Industry Recognition: Awards or certifications can serve as indicators of credibility in the highly competitive market.
Each of these considerations will contribute to a clearer picture of which vendors can be trusted to deliver on their promises.
Conducting Trials and Demos
Finally, conducting trials and demos of selected tools is an invaluable step in the evaluation process. Hands-on experiences allow organizations to gauge functionality and ease of use before committing to a purchase.
- Trial Duration: Ensure that the trial period is sufficient to fully explore the tool’s capabilities. A common duration may be between 14 to 30 days.
- Support and Resources: During demos or trials, assess the level of customer support provided. This includes training materials, FAQs, or direct support options.
- Feedback Mechanisms: Implement a method for collecting feedback from team members involved in the trial. This input is crucial for making an informed final decision.
By effectively utilizing trials and demos, organizations can reduce uncertainty and select a discovery data solution that truly meets their needs.
"A well-rounded evaluation process is key to unlocking the benefits of discovery data tools."
Employing these best practices will allow organizations to navigate the complexities of discovery data pricing, ensuring that the tools adopted contribute positively to their operations.
Future of Discovery Data Pricing
Understanding the future of discovery data pricing is imperative for organizations aiming to navigate the ever-changing landscape of data management. With the increasing reliance on data-driven decisions, pricing models are becoming more complex and diverse. This section explores emerging trends and possible regulatory impacts that organizations must consider when planning their data strategy.
Predicted Trends and Innovations
Several trends are shaping the future of discovery data pricing. First, there is a growing emphasis on flexibility in pricing models. Companies are increasingly favoring subscription-based models rather than traditional one-time payments. This shift allows businesses to adjust their expenses based on changing data needs.
Furthermore, artificial intelligence and machine learning are expected to play pivotal roles. As these technologies advance, they will facilitate more accurate pricing strategies, often linking costs to actual usage metrics. For instance, companies like Microsoft and Google are integrating AI capabilities into their offerings, potentially lowering costs through smarter data alignment.
Another key innovation is the integration of advanced analytics in pricing models. This enables providers to offer tiered pricing, with packages catering to varying levels of data sophistication. For example, smaller businesses might prefer basic packages at lower costs, while larger enterprises could select comprehensive solutions with advanced features.
"In the future, pricing for discovery data tools is likely to reflect not just supply and demand but also the sophistication of the user’s data needs."
Potential Impact of Regulations
Regulations are increasingly critical in shaping discovery data pricing. Governments worldwide are enacting data protection laws, such as the General Data Protection Regulation (GDPR) in Europe, which mandates strict compliance requirements for data handling. Compliance with such regulations often leads to increased operational costs for providers, which may result in higher prices for end-users.
Moreover, the regulatory environment pushes data vendors to enhance transparency in their pricing structures. As organizations face scrutiny over compliance, they will demand clear, unambiguous pricing, reducing hidden fees and strengthening consumer trust. This trend will likely force new entrants in the market to adopt straightforward pricing models to compete effectively.
The End and Recommendations
The conclusion serves a crucial role in tying together the insights presented throughout this article on discovery data pricing. This section highlights the essential elements that readers should retain, offering a clear synthesis of information. In addition, it provides actionable recommendations that empower decision-makers in their quest for the most suitable data solutions. By summarizing key points and providing practical guidance, this section enhances the article's overall value.
Summary of Key Insights
Continuing from previous sections, it is important to distill the core insights into a compact form. The discussion on various pricing models reveals that choices are influenced by factors such as user needs, data complexity, and vendor offerings. The current trends indicate an increasing demand for scalable solutions that adapt to the fast-changing digital landscape. Readers should note the following key insights:
- Diverse Pricing Models: Companies can choose between fixed, variable, and hybrid models, depending on their operational requirements.
- Influence of Data Volume: The cost of discovery data directly correlates with the volume of data processed and the complexity of its structures.
- Growing Importance of AI: AI-driven tools are gaining traction as they offer enhanced capabilities in data analysis and management.
- Vendor Credibility: The reliability of the vendor plays a significant role in successful data integration and ongoing support.
Guidance for Decision-Makers
In navigating the complex arena of discovery data pricing, decision-makers must approach the task with a structured mindset. Here are some recommended steps:
- Conduct a Detailed Requirements Analysis: Start by assessing internal needs. Determine what data is vital for business operations and how much should be invested in data discovery tools.
- Evaluate Cost-Effectiveness: Consider not only the upfront costs, but also the long-term economic impact. Are the tools likely to provide a positive ROI? Analyze different pricing models thoroughly before making a decision.
- Prioritize Vendor Reliability: Research and assess different vendors thoroughly. Look for case studies, reviews, and testimonials. Prioritize vendors that demonstrate a history of reliability in customer service and technical support.
- Engage in Trials: Before committing, take advantage of free trials or demos. This practice enables a hands-on evaluation of how well different tools align with business needs.
- Stay Informed on Trends: Keep an eye on emerging technologies and regulations that could affect discovery data costs. By staying current, decision-makers can adapt their strategies effectively.
By concentrating on these areas, professionals can make informed decisions that not only optimize costs but also enhance data-driven strategies.