Top Free Statistical Software for Mac Users in 2023
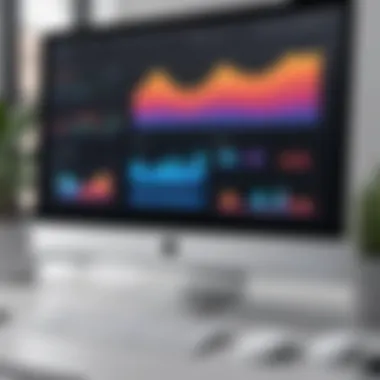
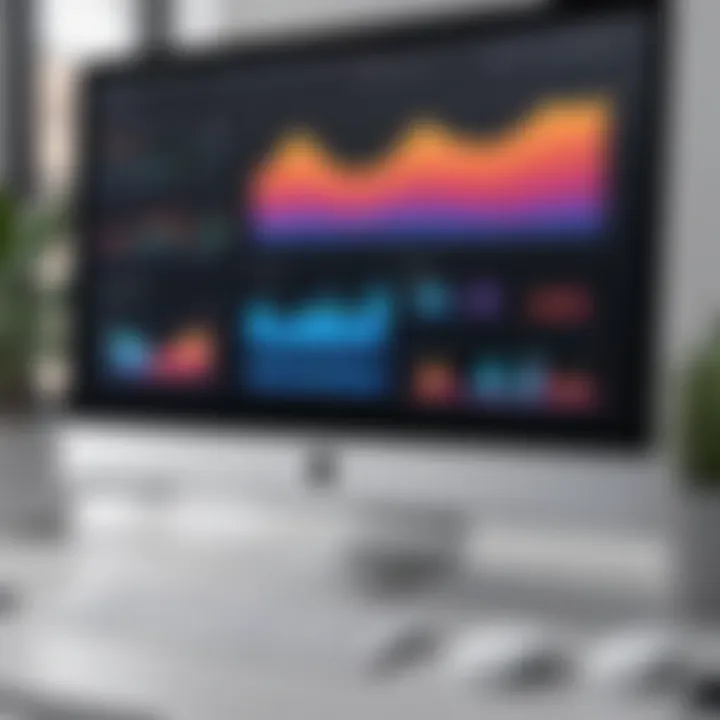
Intro
In an age where data reigns supreme, being equipped with the right tools can make all the difference. The emergence of free statistical software is a game changer, particularly for those using Mac computers. These software options democratize data analysis, allowing a wide audience—ranging from students and freelancers to seasoned professionals—to delve into data without breaking the bank.
This article aims to dissect the free statistical software landscape for Mac users, offering guidance on selecting the most suitable option based on individual needs. We will explore features, advantages, and potential drawbacks of various programs while highlighting ideal scenarios for use. As we navigate this realm, we hope to empower readers to harness the capabilities of data analysis effectively.
Software Needs Assessment
Before diving headfirst into the rich tapestry of software options, it is vital to pinpoint what one actually requires. Just like a chef wouldn’t use a frying pan when a grill is needed, identifying user requirements is paramount.
Identifying User Requirements
First things first, what do you intend to achieve with statistical software? Some key considerations might include:
- Nature of Data: Are you handling numerical data, categorical variables, or a mix of both?
- Complexity of Analysis: Will basic descriptive stats sufficce, or do you need advanced statistical modeling?
- User Proficiency: Are you an experienced statistician or just dipping your toes into the water?
- Collaboration Needs: Will you be teaming up with others, necessitating the sharing of data or analysis results?
Taking stock of these needs will guide your exploration and ensure you choose a software package that aligns with your objectives.
Evaluating Current Software Solutions
It’s also wise to peek into what’s already out there before making a commitment. The world is brimming with free statistical software options for Mac, each with its own strengths and weaknesses. When evaluating, consider the following:
- User Reviews and Forums: Websites like Reddit host discussions that'll give you candid insights on what to expect from each software.
- Documentation and Tutorials: Check if the software provides comprehensive guides that can help you get up to speed.
- Community Support: A vibrant user community can be a boon for troubleshooting and knowledge-sharing.
Data-Driven Insights
Understanding market trends and software performance can shed light on which tools are suitable for your needs. This insight can influence your decision significantly.
Market Trends Overview
Free statistical software has gained traction among various demographics. Institutions like universities and small businesses are particularly receptive to cost-effective software solutions. Popular programs tend to evolve quickly with user feedback, resulting in frequent updates and enhancements. Keeping an eye on trends can let you anticipate which software may best serve you in the long run.
Performance Metrics
When assessing different software options, looking at performance metrics is essential. Some factors to consider include:
- Speed of Analysis: Does the software handle large datasets without a hitch?
- User Interface: Is the program intuitive, or will you waste time trying to navigate it?
- Integration: Can it work seamlessly with other tools you rely on?
"The right tools can transform raw data into golden insights—don't settle for anything less."
Evaluating these aspects will identify which tool stands out and will keep you from getting bogged down in complicated processes.
In summary, by assessing your needs and understanding the available options, you lay a solid foundation for navigating the landscape of free statistical software for Mac users.
Prolusion to Statistical Software
In today's data-driven world, statistical software plays a vital role in analyzing and interpreting complex datasets. With an increasing number of individuals and businesses relying on data for decision-making, understanding the landscape of statistical software becomes paramount. This article zeroes in on free statistical software options, particularly tailored for Mac users. These tools not only facilitate robust analysis but also foster accessibility for budding analysts and seasoned professionals alike.
Importance of Statistical Analysis
Statistical analysis is the backbone of informed decision-making. Whether in research, business, or public policy, the ability to derive insights from data drives strategies and interventions. It allows for hypothesis testing, predictions, and uncovering trends that might otherwise go unnoticed.
When data speaks, it reveals patterns, correlations, and sometimes surprises that can lead to actionable insights. The implications of these analyses can be profound, impacting everything from economic forecasts to public health initiatives. Being able to navigate through this data landscape is a skill every professional should ideally cultivate. Without the proper instruments for analysis, even the most vast datasets become mere numbers on a page.
An Overview of Software Types
Statistical software comes in various shapes and sizes, each designed to cater to specific needs or industries. Understanding these types can help users better align their selections with their analysis goals. Here’s a quick rundown:
- General-purpose Programs: These software solutions, like R or Python, can handle a variety of statistical methods and are versatile enough to be applied across different disciplines.
- Specialized Software: Tailored for particular fields, these tools might focus on areas such as bioinformatics or econometrics, providing more advanced capabilities in defined contexts.
- User-Friendly Interfaces: For those looking for simplicity, programs like Jamovi present an engaging graphical interface that makes statistical analysis approachable for those who may not be deeply versed in coding or statistical theory.
When selecting software, it is important to think about the type of analysis you need to perform and the level of complexity you're comfortable with.
The main takeaway is that there’s a free option out there for everyone. By familiarizing oneself with the available statistical software options, Mac users can enhance their ability to dissect data and draw meaningful conclusions.
Benefits of Using Free Statistical Software
When it comes to data analysis today, the type of statistical software you use can greatly influence your work's effectiveness and efficiency. Free statistical software provides an avenue for everyone—from students to seasoned professionals—to dive into data without burning a hole in their pockets. In this section, we’ll explore several critical advantages that these free tools offer.
Cost-effectiveness
One of the most apparent benefits of free statistical software is its cost-effectiveness. Unlike priced options that can set users back hundreds or even thousands of dollars, these alternatives fall within a budget-friendly range. For students or small enterprises, every penny matters, and free software can help retain cash for other essential needs.
Moreover, since most of these programs are open-source, they often come with an inclusive community backing that thrives on contributions. This leads to ongoing development, fixing bugs, and achieving features you'd expect from premium solutions.
"Access to resources shouldn’t be just a luxury, and free statistical software ensures that anyone interested in data has the tools they need at their fingertips."
Long-term cost savings also feature significantly. Investing in free software often involves fewer expenses related to licenses and upgrades, which allows users to allocate funds toward other critical business operations or educational pursuits.
Community Support and Resources
Another strong point is the community that springs up around free statistical software. You see, when a lot of folks rally around a tool, support tends to flourish. Users regularly share insights, solutions to common problems, and create tutorials that can greatly expedite your learning process.
Many users turn to forums like reddit.com for discussions surrounding challenges encountered and how they can overcome them. These interactions not only enhance your understanding but also help build networking connections. Things such as customized user guides, video tutorials on platforms like YouTube, and extensive documentation provide novice analysts a kind hand while navigating this complex world of data.
Flexibility and Customization
Free statistical software often offers tremendous flexibility that proprietary software may not. This means users can adapt and tailor the software to better suit their specific requirements. For example, if the existing tools don’t meet a particular need, savvy users can modify the source code—something unheard of in proprietary solutions.
The beauty of this customization lies in its ability to evolve alongside the user. Organizations can add features tailored to their dataset's specificities or even create new algorithms that give them a competitive edge. Furthermore, users have the freedom to work with various data formats, ensuring they can manipulate data in ways that align with their analysis goals.
In sum, the merits of free statistical software extend beyond monetary savings. It fosters a rich ecosystem of community support and adaptable tools that can significantly elevate the quality of data analysis. This accessibility lays a robust foundation for users to engage with data creatively and effectively.
Key Considerations When Choosing Software
When contemplating which statistical software to adopt, several pivotal factors come into play. These considerations are essential for anyone who plans to engage in meaningful data analysis, whether for academic research, business strategy, or personal projects. The right software not only enhances your productivity and efficiency but also directly influences the quality of your analysis. A well-informed decision can set the foundation for successful data exploration and interpretation.
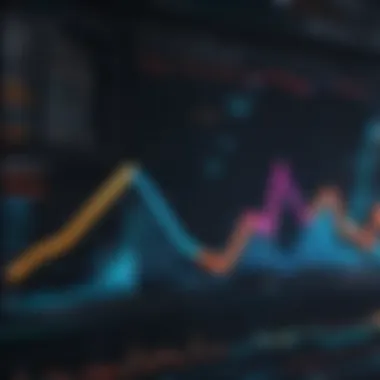
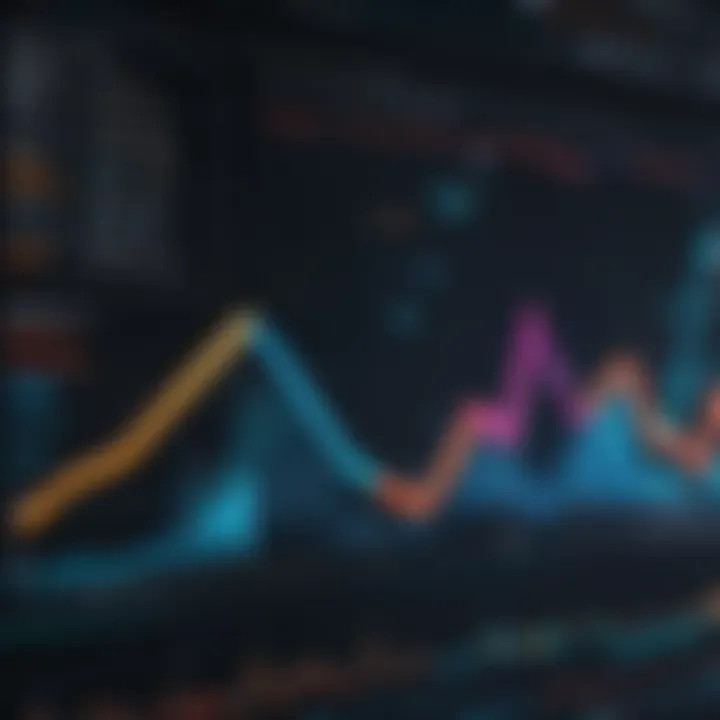
User Interface and Usability
In the realm of statistical software, the user interface often acts as the gateway for the entire experience. An intuitive design can make a world of difference. Software that has a cluttered layout or demands a steep learning curve can quickly become a source of frustration.
- Clarity: The visual elements should be clear and easy to navigate.
- Accessibility: Easy access to frequent tools saves time and alleviates unnecessary stress.
- Help Resources: Good software will have comprehensive support, like tutorials or an active user community.
For example, RStudio is a popular choice among users not just because of its robust capabilities but also due to its user-friendly layout. Navigating its features becomes almost second nature after a short period. This matters a lot, especially when analyzing large datasets.
Statistical Features and Capabilities
The core purpose of any statistical software is to provide a set of tools for analysis. Thus, examining specific features is crucial. Key variables to watch out for include:
- Descriptive statistics: Measures like mean, median, and mode.
- Inferential statistics: T-tests, chi-squared tests, ANOVA, etc.
- Machine learning capabilities: If your analysis may require predictive modeling, ensure the software supports those needed functions.
- Ease of customization: Flexibility can transform a good tool into a great one.
For instance, Python, with libraries like Pandas and SciPy, is potent because of its statistical capabilities alongside an extensive ecosystem of data manipulation functions. It allows users to tackle almost any statistical problem.
Compatibility with Data Formats
Data comes in various formats, and the ability of software to seamlessly integrate with different data types is paramount. Compatibility can greatly affect workflow efficiency. Here are aspects to consider:
- File support: Check whether it can work with Excel spreadsheets, CSV files, or databases.
- Data import/export options: Being able to export results into common formats can facilitate sharing and collaboration.
- API Integration: For more advanced analytics, consider software that can link with other tools or platforms.
For example, Jamovi offers straightforward options for importing diverse file formats, which can enhance collaboration and ease data sharing among teams.
Choosing the right statistical software is not merely a matter of personal preference. An informed choice can lead to richer insights and more reliable outcomes.
Featured Free Statistical Software for Mac
The realm of free statistical software for Mac users is crowded with choices, yet understanding which tool fits best for individual needs is paramount. This section will unpack several standout options, each replete with unique features and advantages that cater to a wide array of statistical analysis requirements. Users effectively arm themselves with knowledge about these tools, making data analysis more approachable and effective.
R and RStudio
Overview of R
R is not just your average programming language; it has become the backbone of statistical computing and graphics. Developed initially in the 1990s, R has gained traction in the data analysis community due to its flexibility and robust capabilities. The open-source nature of R sets it apart from proprietary software, enabling users to delve into statistical techniques without a hefty price tag. Its vast array of packages extends R's functionality, allowing users to perform virtually any statistical analysis they can imagine. A key characteristic is its powerful plotting capabilities, which provide a visual to statistical results, making it a preferred tool for statisticians and data analysts alike. However, the learning curve can be steep for those unacquainted with programming, possibly deterring some users.
Installing R and RStudio
Installing R alongside RStudio, the integrated development environment (IDE) tailored for R, enhances the user experience remarkably. The installation process is straightforward, involving downloading binaries directly from CRAN and RStudio’s website. Users appreciate this simplicity as it allows them to begin their analysis without unnecessary delays. RStudio’s interface offers a user-friendly ecosystem for coding, debugging, and visualizing data results, which is a significant advantage, especially for newcomers. However, some may find it daunting at first glance due to the plethora of options and tools available.
Core Packages and Libraries
The strength of R lies not only in the core language but also in its packages and libraries. These tools expand R's functionality significantly, providing users with resources for everything from linear regression to time-series analysis. Libraries like and have become essential in the toolkit of many analysts, offering enhanced data manipulation and visualization capabilities. The diversity of these packages means that users can tailor R to their specific needs, making it an exceptional choice. However, it's worth noting that maintaining and updating these packages can sometimes be cumbersome for users, requiring a bit of diligence as projects evolve.
Python with Pandas and SciPy
Getting Started with Python
Python is swiftly emerging as a favorite amongst data analysts and scientists for its readability and wide-ranging applications. Getting started with Python involves installing the interpreter and relevant packages like Pandas and SciPy, both integral for statistical analysis. The community support around Python is vast; numerous tutorials and forums exist to help newcomers navigate the initial complexities of coding. Its strong emphasis on clear syntax makes Python more appealing than other programming languages. However, Python can feel overwhelming to complete novices without a programming background, particularly when it comes to setting up an environment.
Data Visualization Tools
When it comes to data visualization, Python offers various libraries like Matplotlib and Seaborn that allow users to create stunning graphics with a few lines of code. These tools support customization and interactivity, making them ideal for data presentations. The capability to visualize data effectively enhances comprehension and draws insights at a glance. However, the initial setup for these libraries can sometimes be tricky, potentially discouraging less tech-savvy users.
Statistical Analysis and Machine Learning
With libraries such as SciPy, SciKit-Learn, and StatsModels at their disposal, Python users can perform detailed statistical analysis and machine learning tasks with ease. The integration of these tools covers a wide array of statistical techniques and algorithms, enabling analysts to explore robust analytical possibilities. This makes Python a versatile option, catering to both those focused on statistical study and those venturing into machine learning. Nevertheless, certain machine learning models might require additional time to master due to their complexity.
PSPP
Prologue to PSPP
PSPP serves as an open-source alternative to SPSS; it is designed for educating users about statistical analysis without the cost associated with proprietary software. Its familiarity to SPSS users makes it an attractive choice for those transitioning from commercial tools. While it may not possess all capabilities of its counterpart, PSPP covers essential analysis features like descriptive statistics, t-tests, and ANOVA. This accessibility makes the program worth considering for educational institutions and researchers looking for a no-cost solution. However, more advanced statistical methods may be limited.
User Navigation
PSPP sport a user-interface reminiscent of SPSS, allowing new users to adapt quickly if they have prior experience with the latter. The straightforward menu options make for easy navigation through various functionalities. Users benefit from a simple point-and-click method, which lowers barriers for entry. However, for those who prefer coding over GUI interactions, PSPP may feel somewhat restrictive.
Key Functionalities Comparison with SPSS
While PSPP aims to replicate many features of SPSS, it's crucial to understand its limitations as well. The software supports a good range of statistical tests and procedures, making it suitable for general analysis needs. Users can perform most basic tasks like frequency distributions, regressions, and other statistical tests found in SPSS. However, advanced users might find missing features in PSPP that are present in SPSS, which could inhibit more complex analyses.
Jamovi
User-Friendly Interface
Jamovi prides itself on having a clean, modern interface that feels intuitive even for first-time users. The platform is set up to allow users to execute statistical analyses without needing extensive programming knowledge. What's particularly beneficial is the drag-and-drop functionality that facilitates data import and manipulation. For professionals who are pressed for time, this ease of use can be tremendously appealing. However, advanced users may find the simplicity limiting for more complex statistical tasks.
Statistical Analysis Features
The statistical features of Jamovi encompass a broad array of analyses, from t-tests to ANOVA and regression models. Jamovi also allows for real-time data analysis as users modify their datasets, which enhances workflow efficiency. This coupling of statistical power with user-friendliness makes it a strong candidate for novice and intermediate analysts alike. However, it may lack some of the intricate analytical capabilities found in open-source alternatives like R.
Community Resources and Extensions
Community engagement in Jamovi is noteworthy, with resources ranging from user forums to tutorials available for deep dives into its capabilities. Developers frequently contribute extensions that expand Jamovi's functionality, ensuring that users have access to cutting-edge statistical methods. The existence of a supportive community bolsters the user experience significantly, yet the reliance on community contributions might make some features less stable or fully polished compared to more established software solutions.
JASP
Ease of Use and Features
JASP comes equipped with an inviting interface that lowers the entry barrier for statistical analysis. Its aesthetically pleasing layout is designed to deliver statistical results simply and directly, which is significantly beneficial for users who may feel overwhelmed by more complex programs. Not only is the interface user-friendly, but JASP also offers comprehensive analysis options ranging from basic to advanced statistical techniques. However, some users may wish for more advanced customization options that other statistical software provides.
Bayesian Statistics
JASP places a strong emphasis on Bayesian statistics, which differentiates it from many other free statistical tools. For researchers who are keen on exploring this growing field of statistics, JASP’s user-friendly approach to Bayesian analysis makes it a practical choice. Accessible tutorials and documentation support this, simplifying a complex subject for many. On the downside, the focus on Bayesian methods might alienate users who are accustomed to traditional frequentist analysis.
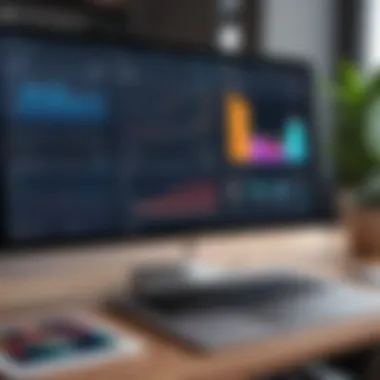
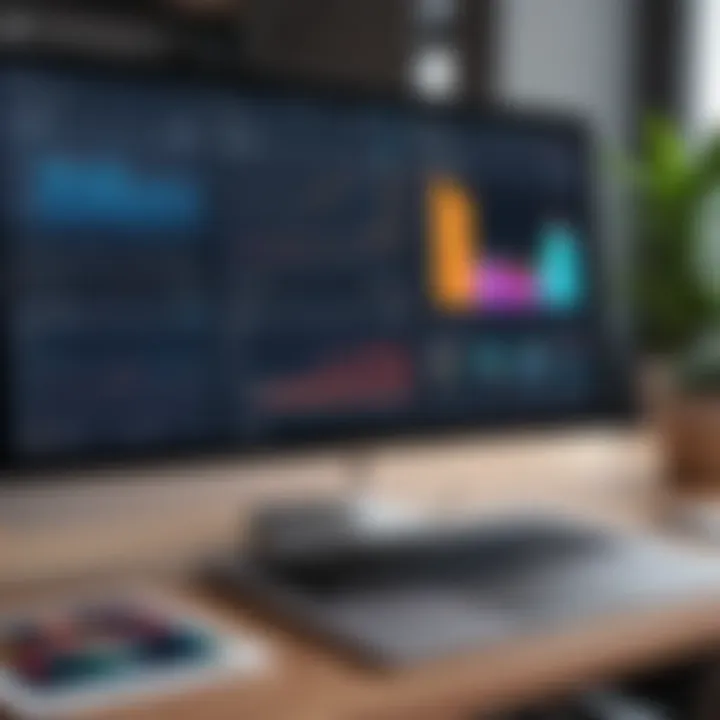
Graphical Output Options
JASP excels in providing rich graphical representations of results, allowing users to visualize their data intuitively. The software automatically generates plots and tables based on analysis conducted, enabling more accessible interpretation of statistical findings. This feature is an alluring draw for users who must present data visually. However, its automatic nature can sometimes lead to unanticipated outcomes, necessitating a careful examination of all outputs.
Installation and Setup Guide
The Installation and Setup Guide is vital in this discussion about free statistical software for Mac users. Getting started with any software often dictates the user experience and can make or break your analytical journey. Proper installation ensures that the software runs smoothly, avoiding any headaches down the line. Moreover, understanding the configuration and the initial protocols allows users to harness the full power of the tools at their disposal.
System Requirements
Before diving into the installation of statistical software, it is essential to check the system's compatibility. Each software comes with its own requirements that one should heed carefully:
- Operating System: Most statistical software options are compatible with the latest versions of macOS. It's crucial to have an updated system to avoid any compatibility issues.
- RAM: Depending on the complexity of the analysis, a minimum of 4GB RAM is often necessary. However, for more intensive operations, especially machine learning tasks, 8GB or more is recommended.
- Disk Space: Ensure you have adequate space for the software itself and additional data sets. This could range from 1GB to several gigabytes, especially if extra packages or libraries are installed.
- Processor: A modern multi-core processor can speed up computations, which is beneficial when working with large datasets.
Understanding these basic requirements is the first step towards a successful installation.
Step-by-Step Installation Process
Once the system checks out, the actual installation process is crucial to setting up your statistical software correctly. Here’s a streamlined process that might vary slightly between different software but generally follows these steps:
- Download the Installer: Find the official website of the software (like R or Python) and download the Mac version of the installer. Be cautious—always opt for the latest stable version.
- Open the Installer: Locate the downloaded file in your Downloads folder, and double-click it to run. This will typically open a new window guiding you through the installation.
- Follow the Prompts: Follow the on-screen instructions. This usually involves agreeing to the terms of service and selecting installation preferences such as the destination folder.
- Complete the Installation: After clicking install, wait for the software to be set up. This might take a few minutes. Once done, you will often receive a prompt stating that installation is successful.
- Launch the Software: Find the software in your Applications folder and launch it. This part marks the beginning of your analytical work.
Initial Configuration and Tutorials
Once installed, the first thing a user wants to do is configure the software to fit personal needs. Here’s how to make the most out of your initial setup:
- Initial Configuration: Some software will ask for some initial settings. Set your preferences such as the default directories for data files or output files. This can save time later.
- Recommended Packages: Many statistical tools allow the installation of additional packages. Familiarize yourself with the core packages to enhance the functionality of the software. For instance, in R, packages like for data visualization or for data wrangling can be vital.
- Tutorial Resources: Leverage the multitude of tutorials available online. Platforms such as Wikipedia, forums on Reddit, or video tutorials on YouTube can serve as excellent starting points for learning the software.
Going through these steps not only sets the groundwork for effective analysis but also boosts confidence for users, especially those new to statistical software.
Statistical Analysis Techniques Using Free Software
In the world of data analysis, the techniques employed can determine the outcomes of extensive research or business insights. This section discusses different statistical analysis techniques available through various free software options on Mac. Engaging with these techniques allows users to make informed decisions, derive meaning from datasets, and ultimately drive effective conclusions.
Data analysis techniques range from basic descriptive statistics to complex inferential methods and machine learning approaches. The advantage of using free statistical software is twofold. Not only does it eliminate the financial barrier that often restricts access to quality analytics tools, but it also opens up a world of possibilities for data exploration. Users can refine their skills without the pressure of costly software, allowing for an experiential learning curve that can adapt to individual needs.
Descriptive Statistics
Descriptive statistics lay the groundwork for data understanding. They provide snapshots of the data at hand and help to summarize characteristics. This method includes calculating measures like mean, median, mode, range, and standard deviation. For instance, using R, users can quickly grab a glimpse of the basic stats with a simple command:
Such a command generates a plethora of information that encapsulates the dataset's features succinctly. Understanding these statistics can guide researchers toward acknowledgment of trends or patterns. Moreover, embracing graphical representations, such as histograms and box plots, enhances the interpretative nature of these statistics. The beauty of descriptive statistics lies in its straightforwardness—helping users see the bigger picture while enabling them to identify outliers that could skew the interpretations.
Inferential Statistics
Moving beyond mere descriptions, inferential statistics allow users to make predictions and draw conclusions based on sample data. This technique is all about making inferences about a larger population using a smaller set. Free software like Python's Statsmodels or R can compute confidence intervals, hypothesis tests, and regression analysis. For example, a confident researcher might postulate a hypothesis like this:
"The average score of a group of students is significantly different from a known benchmark."
After gathering data from a representative sample, one could utilize a simple t-test:
The result provides insights into whether to reject or accept the null hypothesis, thus molding the foundation of analytical conclusions. Inferential statistics hold tremendous value for business professionals who often rely on sampling to make accurate forecasts about market trends, customer preferences, and more.
Machine Learning Approaches
In this digital age, machine learning represents the new frontier for analytical techniques. Leveraging free software tools opens doors to predictive modeling and pattern recognition, driving business intelligence further. With libraries such as scikit-learn in Python, or caret in R, users can easily embark on journeys into classification, regression, and clustering.
Using machine learning allows for advanced data manipulation, which traditionally required expensive software solutions. By tapping into these models, users can gain valuable insights into complex datasets and discover trends that human interpretation might overlook. For instance, segmenting customers based on their purchasing behavior can lead to targeted marketing strategies.
Ultimately, the tools provided by free statistical software make these advanced techniques accessible, allowing analysts to tackle real-world problems with robust methodologies.
Unlocking the potential of statistical analysis through free software enables individuals to navigate their analytical undertakings with confidence and effectiveness. Thus, understanding these techniques equips users to handle a spectrum of data, fostering a sharper understanding of the underlying messages that numbers convey.
Real-World Applications of Statistical Software
Statistical software applications stretch far beyond the confines of academic exercises. The ability to analyze data efficiently opens doors in various domains, providing insights that drive decisions, influence policies, and enhance operations. Understanding how these tools integrate into everyday processes can significantly empower users, especially those navigating their professional or academic journeys. Whether it’s in research, business, or public health, statistical software serves as the backbone for effective data management and exploration.
Research and Academia
In the realm of research, statistical software is indispensable. Scholars rely on these tools to analyze experimental data, test hypotheses, and extract meaningful conclusions from complex datasets. The role of statistical analysis in academia cannot be understated—it’s essential for ensuring that research findings are reliable and reproducible.
For instance, a researcher conducting a clinical trial needs to apply statistical methods to determine if their treatment truly works or if the observed outcomes are due to chance. Here, software like R or JASP becomes crucial. By employing these tools, researchers can not only run tests but also visualize results through graphs and charts, enhancing the clarity of their reports. This proficiency with statistical software enhances a researcher's credibility, enabling them to present their findings to peers or policy-makers compellingly.
Moreover, statistical analysis techniques enable students to gain practical skills. Graduate programs often require coursework that incorporates hands-on software use, helping students transition smoothly from theory to practice. As they deepen their understanding of topics such as regression analysis or ANOVA, they also bolster their statistical literacy—a skill highly valued across various career paths.
Business Analytics
The landscape of business decision-making has evolved tremendously, thanks to statistical software. Companies today are inundated with data, and those armed with robust analytical tools can transform raw numbers into actionable insights. This capability isn't just advantageous; it's often the difference between thriving or merely surviving in a competitive market.
Consider a retail chain that utilizes Python with Pandas to analyze customer purchasing patterns. By examining data, businesses can identify trends, forecast sales, and tailor marketing strategies—all grounded in statistical evidence.
Additionally, predictive analytics powered by software like R can enhance customer targeting. Take, for example, a campaign geared towards customer retention; using statistical models, businesses can analyze prior purchasing behaviors and predict which customers are likely to churn, thereby allowing timely interventions.
The implications of applying statistical software in business analytics are extensive. It streams decision-making processes, calibrates strategies based on data, and amplifies efficiency, ultimately leading to improved business outcomes.
Public Health Studies
In the field of public health, the stakes are high, and the impact of statistical software resonates deeply. Health professionals leverage these tools to analyze data related to disease outbreaks, treatment efficacy, and health surveys. For example, during an epidemic, public health officials must analyze vast amounts of data to understand transmission rates, the effectiveness of health interventions, and demographic impacts.
Software like PSPP allows researchers to perform complex analyses like regression modeling or survival analysis without the financial barriers posed by commercial options. Such analyses can lead to policy changes that potentially save lives.
The ability to visualize data trends aids health communicators as well. When presenting studies on vaccine effectiveness, for instance, graphical representations of the data can convey information much more clearly than raw numbers alone. This clarity is essential for public understanding and compliance.
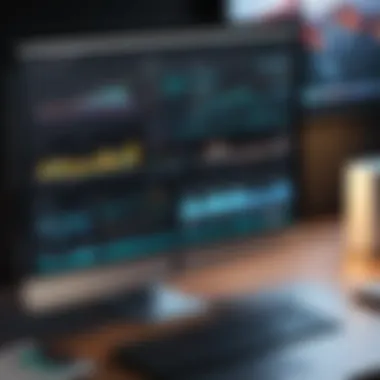
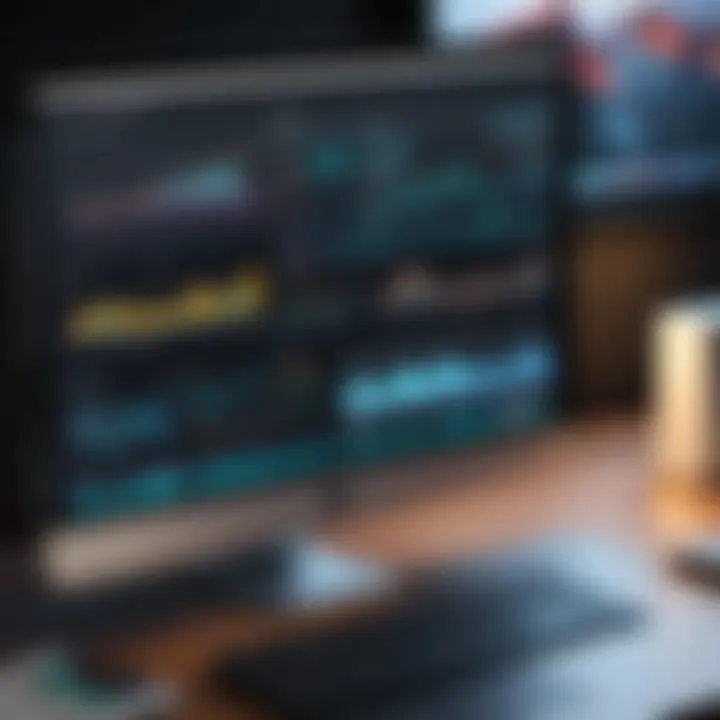
Furthermore, public health sectors often collaborate with academic institutions. Those with experience in statistical software can more easily bridge the gap between research findings and on-the-ground practices—ultimately making communities healthier.
Statistical software bridges the gap between raw data and powerful insights that can transform industries and improve lives.
In summary, the real-world applications of statistical software are vast and varied. From propelling academic research forward to sharpening business strategies and enhancing public health initiatives, these tools are not just accessories but foundational instruments in today’s data-driven world.
Challenges and Limitations
Although the advancement of free statistical software may seem like a game changer in the field of data analysis, it’s not all sunshine and rainbows. Understanding the challenges and limitations of these tools is crucial. Every software comes with its set of nuances that can impact the user experience, especially for those diving into statistical analysis for the first time.
Learning Curve for New Users
Getting a handle on statistical software can oftentimes feel like learning to ride a bike uphill. New users may face a steep learning curve, which can hinder their ability to effectively analyze data right out of the gate. While the user-friendly interfaces of some software like Jamovi present an accessible entry point, others like R could leave beginners scratching their heads.
The common experience is that many users struggle with commands, scripts, or even navigating through complex menus. A somewhat bewildering experience can arise as jargon-filled documentation often adds to confusion. The meticulous details in statistical methods also require a level of understanding that many newcomers might not possess. Consequently, newcomers can feel a bit overwhelmed, urging them to break out into cold sweats, especially when deadlines loom.
Despite these hurdles, the key to mastering these tools lies in practice and patience, plus the wealth of resources available online for learning. Ultimately, persistence can pave the way to competence.
Limited Support for Advanced Techniques
One notable drawback of using free software is the narrow range of support it tends to provide for advanced statistical techniques. Users who need to deploy sophisticated models might discover that certain features are either absent or not up to snuff compared to paid alternatives.
Tools like JASP and PSPP cover essential analysis options, yet they can lack depth in areas such as advanced regression or machine learning algorithms. This limitation can prompt frustration among users looking to push the boundaries of their analytics capabilities. While the software may offer ample utility for basic tasks, serious analysts might find themselves biting the bullet and needing to switch to paid software if they require specialized assistance.
To mitigate these issues, users should assess their specific analytical needs before diving in, keeping in mind that while the software might cater to introductory learners, advanced tasks might necessitate deeper investment.
Performance Issues on Older Systems
Performance can be another thorny issue, especially for users operating on older Mac systems. Many free statistical software options demand a significant amount of computing power to run efficiently. So, if your machine is showing signs of age, you might find these tools sluggish, leading to longer processing times and less productivity.
Users with limited RAM or older processors might endure unexpected crashes while juggling large datasets, which can be a major hassle. If your Mac struggles with multitasking apps already, running resource-intensive statistical software could easily turn into a treadmill of disappointment.
However, it's not all doom and gloom. Optimizing settings, closing unnecessary applications, and periodically updating your software can help alleviate some of these performance hiccups. But, do remember that old tech has its limitations, and at some point, an upgrade may be in order for those who aim to work seamlessly with statistical analyses.
"Understanding the limitations of free statistical software is not just about overcoming obstacles, but also about making informed decisions to enhance data analysis effectively."
Future Outlook of Statistical Software
The realm of statistical software is in a constant state of evolution, driven by technological advancements and user needs. Understanding the future outlook of statistical software is crucial for Mac users, as it not only influences choices today but also shapes the direction of data analysis in the coming years. This section delves into the emerging trends, the role of cloud technologies, and the dynamic evolution of open-source communities.
Emerging Trends in Data Analysis
As data becomes an increasingly valuable asset across various fields, several trends are shaping the way analysis is conducted. Firstly, there's a growing emphasis on real-time analytics. Users want to draw insights from data as it streams in, making traditional batch processing somewhat obsolete for time-sensitive decisions. Alongside this, automated data analysis, driven by machine learning algorithms, is gaining traction. Automation allows users to analyze vast data sets without getting lost in complexity.
Also worth mentioning is the rise of visual analytics. Users are favoring tools that let them visualize data interactively. The push towards user-friendly interfaces means even those who aren’t data scientists can grasp and manipulate data effectively. Additionally, the intersection of data analysis with Artificial Intelligence is becoming increasingly critical. AI is not only serving as a powerful analytical tool but is also pushing the boundaries of what statistical software can achieve.
"The fusion of data science and AI is reshaping how we perceive and interact with data."
Integration with Cloud Technologies
The transition to the cloud can no longer be ignored in discussions of statistical software's future. Cloud technologies offer flexibility and scalability that local software installations often struggle to match. This evolution allows users to access tools and data from anywhere, at any time, significantly enhancing collaboration among teams located in different places. For businesses, cloud integration can streamline processes like data storage and sharing, reducing the need for substantial hardware investments.
Moreover, cloud environments often host robust computational power, enabling users to run complex analyses that might otherwise slow down their local machines. As such, merging statistical software with cloud platforms fosters a more collaborative ecosystem, where community contributions can be immediately integrated and shared. This can also lead to a lower barrier to entry for new users, as they can start analyzing data without heavy initial investments in software or hardware.
Evolution of Open Source Community Contributions
The open-source movement is vital in shaping the future of statistical software. Contributions from dedicated developers and users lead to rapid advancements and flexibility in functionalities. Open-source software allows users to foster growth through community collaboration, often translating into quicker updates, bug fixes, and new features driven by actual user needs.
Moreover, the growth of online communities, such as forums on platforms like Reddit, signifies a shift towards collective learning. Users can now easily share insights, code snippets, and methods, enhancing the overall knowledge base. The community spirit can also boost customizations where software evolves according to niche requirements across various industries. Consequently, open-source contributions continue to enhance accessibility, pushing the envelope on what's possible in data analysis and ensuring users always have cutting-edge tools at their disposal.
End
As we reach the end of our exploration into free statistical software for Mac users, it's crucial to recognize the significance of this topic in today’s data-driven landscape. The ability to analyze and interpret data is not just an asset; it's increasingly becoming a necessity across various sectors, from academia to business and public health. By utilizing free statistical software, users can access powerful tools that were once reserved for those with deep pockets or advanced training, effectively democratizing data analysis.
The benefits of opting for free software include substantial cost savings, which can be especially advantageous for students and small businesses operating on tight budgets. Furthermore, many of these solutions offer active community support, ensuring that users can find resources and assistance to overcome obstacles as they learn how to use the software more effectively. This sense of community can foster collaboration and innovation, as users share insights and recommend improvements, creating a vibrant ecosystem around the software itself.
Summary of Key Points
- Accessibility: Free statistical software brings advanced analytics capabilities to a wider audience, breaking down financial barriers and enabling more people to leverage statistical insights.
- Cost-effectiveness: Users save on licensing fees that are typical with commercial software, allowing budgets to be redirected to other essential areas.
- Community and Resources: With a wealth of tutorials, forums, and extensions available, the learning curve can be navigated more smoothly. Engaging with other users can foster a deeper understanding of the software and its applications.
- Flexibility: Many of these programs can be tailored to meet specific needs, whether one is inclined towards basic statistics or is delving into more complex analyses, which aids in making the software more relevant to user scenarios.
- Challenges: While free software presents a myriad of benefits, users may encounter limitations regarding advanced features or speed, especially on less powerful devices. Understanding these nuances is essential for optimizing performance.
Further Reading and Resources
In the realm of statistical software, having a go-to collection of resources can greatly enhance your understanding and application of these tools. Free statistical software can be vast and sometimes overwhelming, especially for Mac users who wish to harness its full potential. Thus, this section is dedicated to providing key materials for further reading and exploration in various formats, catering to those who seek to deepen their statistical know-how.
Recommended Books
Books form the bedrock of knowledge. While free software allows experimentation, reading well-curated texts can be a game changer. Here are a few titles worth considering:
- "The Art of R Programming" by Norman Matloff - This book serves as an introductory guide for R, perfect for novices who want more than just a surface understanding. It builds a solid foundation and dives into practical examples that illustrate key statistical concepts.
- "Python for Data Analysis" by Wes McKinney - Written by the creator of Pandas, this book is crucial for anyone using Python. It focuses on practical data analysis using Python and is stuffed with clear explanations and well-structured examples to guide you.
- "Discovering Statistics Using R" by Andy Field - Well known in academic circles, this book makes the subject approachable and engaging while drawing real-world connections to statistical methods.
These books not only equip users with skills but also encourage critical thinking about data and methodologies in analysis.
Online Courses and Tutorials
Online learning platforms have exploded in recent years, allowing flexible learning that fits into anyone's schedule. They offer a hands-on approach to mastering statistical software. Here are some great options:
- Coursera - Offers courses specifically on R and Python for data analysis. Courses range from beginner to advanced, often taught by university professors.
- edX - Another platform where you can find lectures from prestigious universities covering a wide array of statistical topics.
- YouTube - Surprisingly valuable. Channels that focus on data science and statistics cover R and Python tutorials in bite-sized videos, making it easier to digest complex concepts.
Engaging with online tutorials enriches practical knowledge and can provide unexpected insights, making them a worthy investment of time.
Helpful Forums and Community Groups
Communities around statistical software often harbor gems of information. These forums serve as platforms for both querying and sharing knowledge. Consider participating in these hubs:
- Reddit - Subreddits like r/statistics and r/datascience are bustling with discussions and problem-solving threads. You can tap into a wealth of knowledge from amateur enthusiasts to seasoned professionals.
- Stack Overflow - When you're stuck, there's a high chance that someone has asked your question before. It’s a go-to platform for specific issues about R, Python, and other statistical tools.
- Facebook Groups - Many statistical communities exist where members share resources, tips, and advice. Look for groups centered on R, Python, or any other software pertinent to your analysis.
"Peer discussions can tilt your understanding from narrow to broad in a matter of moments."
Engaging with these communities not only solves immediate queries but also puts you in contact with the latest trends and techniques in statistical analysis.
In essence, further reading and resources are indispensable for anyone serious about statistical software. They foster a deeper understanding of tools and techniques, enhancing one's capability to analyze data effectively. Whether you prefer reading books, taking courses, or engaging with fellow users in forums, these resources are pillars supporting your journey in the world of data.