Collibra Data Profiling: Mechanisms and Applications
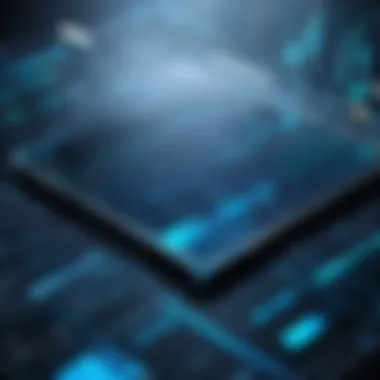
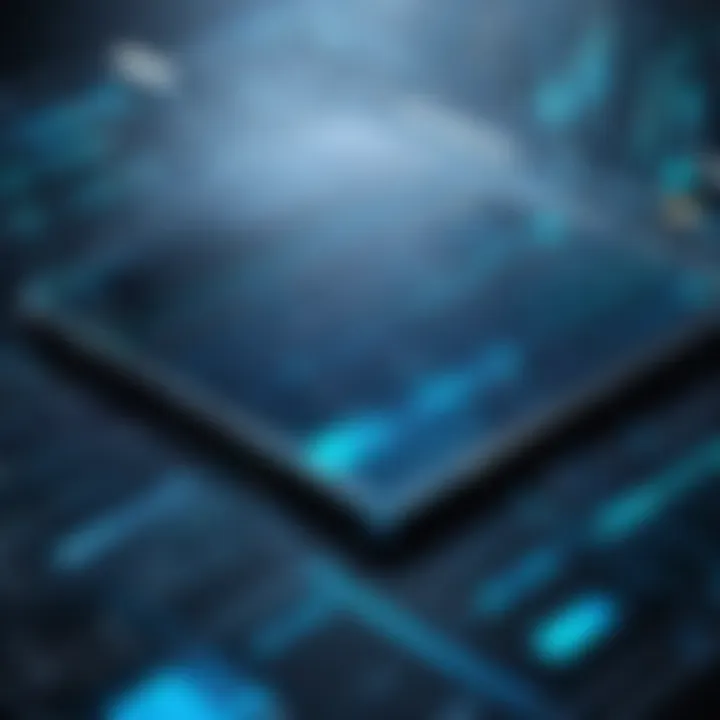
Intro
Data profiling stands as a foundational activity within the realm of data management, serving to understand and enhance the quality of data. When implemented through Collibra, a leading data governance platform, data profiling assumes crucial importance. It provides tools that guide organizations in assessing their data assets effectively. This assessment ultimately supports better decision-making and drives more strategic business outcomes.
Understanding Collibra's methodologies and applications is essential for data professionals aiming to maintain high standards of data quality within their organizations. Improved data quality enables organizations to leverage insights gleaned from their data, thereby optimizing operational efficiencies and supporting regulatory compliance. Through data profiling, businesses can embrace a proactive stance on data governance, identifying anomalies and areas for improvement.
In the following sections, we will explore the necessity of a software needs assessment, the integration of data-driven insights, and the practical applications of Collibra within data governance frameworks. Let's begin by assessing the software requirements that underpin effective data profiling practices.
Preamble to Collibra Data Profiling
In today’s data-driven environment, understanding how to assess and manage data is crucial. Collibra Data Profiling is a key tool that organizations can leverage to enhance data quality and governance. The importance of this process lies in its ability to provide insights into the structure, quality, and consistency of data within a system. Effectively deploying data profiling can lead to better decision-making and greater efficiency in data management practices.
What is Data Profiling?
Data profiling is the process of examining data from existing sources and collecting statistics and informative summaries about that data. This process plays a vital role in understanding the condition of data integration, evaluation, and cleansing efforts. Data profiling involves a series of techniques such as:
- Structure Analysis: Identifying the format of data and its structures, for instance, checking if a numeric field contains only numbers.
- Content Analysis: Evaluating the actual data values for issues like missing values, outliers, and distributions.
- Relationship Analysis: Understanding how different data elements relate to one another.
By employing these techniques, organizations can identify data quality issues before they affect operations, thus allowing for timely interventions.
Overview of Collibra
Collibra is a leading data intelligence platform designed to help organizations manage their data governance, data quality, and compliance. It provides a comprehensive environment for data stewards and business users to collaborate effectively. Key functionalities include:
- Data Cataloging: Collibra enables users to easily find, understand, and govern their data assets.
- Workflow Automation: This feature streamlines data governance processes, allowing for more efficient data management.
- Collaboration Tools: Users can work together to solve data issues, thereby increasing accountability and data ownership.
- Data Quality Monitoring: Collibra provides tools to continuously monitor and improve data quality across the system.
Mechanisms of Data Profiling in Collibra
Data profiling is a critical component in ensuring that organizations manage their data effectively. Within the realm of Collibra, data profiling mechanisms serve as the backbone for evaluating data quality issues and optimizing data management processes. Understanding how Collibra implements these mechanisms can prove invaluable for professionals aiming to leverage data for improved insights and decision-making.
Understanding Profiling Techniques
Profiling techniques in Collibra are formulated to systematically assess dataset attributes, encompassing both quantitative and qualitative analyses. These techniques help identify anomalies, inconsistencies, or redundancies within data sets. Key profiling techniques include:
- Structure Analysis: This entails examining the format and structure of the data, ensuring that it adheres to predefined standards. It helps in detecting structural errors and ensuring data integrity.
- Content Analysis: This technique evaluates the actual data entries, identifying patterns, distributions, and potential outliers. By analyzing content, users can gain insights into the data set's overall quality and usability.
- Relationship Analysis: In Collibra, this involves assessing the interconnections between different data entities. It helps in understanding data dependencies and can unveil hidden relationships that impact data value.
By employing such techniques, organizations can develop a clearer understanding of their data landscape, thus enhancing their capacity to utilize data strategically.
Key Features of Collibra Data Profiling
Collibra offers several key features that streamline the data profiling process, making it a preferred choice for businesses focused on data governance. Some of these features include:
- Automated Profiling: Collibra allows for automated data profiling tasks, reducing manual effort and increasing efficiency. Users can schedule regular profiling to ensure ongoing data quality monitoring.
- Customizable Dashboards: Users can create tailored dashboards to visualize profiling results, making it easier to track data quality metrics and trends over time.
- Integrations with Other Tools: Collibra's profiling mechanisms seamlessly integrate with various data management tools, including ETL (Extract, Transform, Load) processes. This ensures that data quality is maintained throughout the data lifecycle.
- Collaboration Features: Data profiling results in Collibra can be shared across departments. This collaborative approach fosters a culture of data stewardship, where everyone is accountable for data quality.
"Effective data profiling in Collibra lays the groundwork for informed decision-making and robust governance strategies."
These features collectively enhance the capability of organizations to maintain high data quality and to leverage data effectively, reinforcing the importance of data profiling as a vital mechanism in data governance.
Benefits of Using Collibra Data Profiling
Collibra Data Profiling presents various advantages for organizations aiming to enhance their data practices. In today’s data-driven world, the integrity and usability of data are paramount. This section discusses three core benefits derived from using Collibra Data Profiling: enhanced data quality, improved decision-making, and increased efficiency in data management. Each benefit is crucial for businesses to operate effectively, and understanding these elements is essential for any organization working with large data sets.
Enhanced Data Quality
A fundamental benefit of utilizing Collibra Data Profiling is the significant improvement in data quality. Data quality refers to the accuracy, completeness, and reliability of data, which ultimately affects trust in the information used across different departments. By applying Collibra’s profiling tools, organizations can systematically assess and monitor their data. This involves identifying anomalies, detecting inaccuracies, and ensuring consistent data formats. As a result, businesses can rely on data that is not only correct but also timely.
To maximize data quality, it is essential to implement continuous profiling. This process creates a feedback loop that maintains data integrity over time. Problems can be addressed before they escalate, allowing organizations to tackle issues proactively. Enhanced data quality not only boosts operational efficiency but also increases the overall confidence of stakeholders in data-driven decisions.
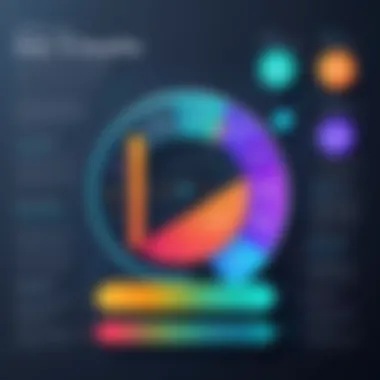
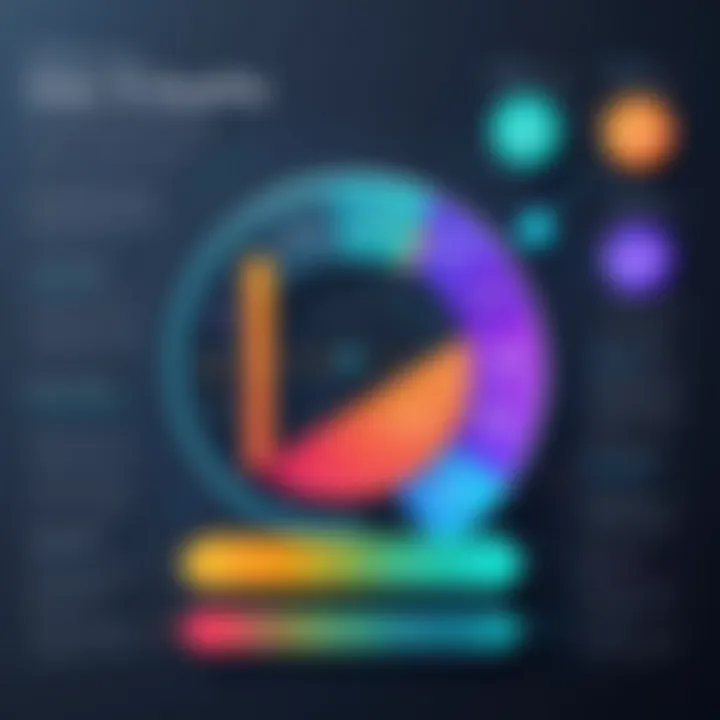
Improved Decision-Making
Collibra Data Profiling aids in honing decision-making processes through better data visibility and reliability. Accurate data serves as a strong foundation for informed decision-making. When data is profiled correctly, it informs executives and managers about trends, opportunities, and potential risks within operations.
Moreover, collated data from various sources can provide a comprehensive view of the business landscape. Collibra's data profiling enables users to integrate insights from disparate datasets seamlessly. This integration leads to refined analytical capabilities. By leveraging reliable insights, organizations can enhance their strategic planning efforts. This improved foresight helps in capitalizing on opportunities more efficiently.
Increased Efficiency in Data Management
Increased efficiency in data management is another significant benefit of using Collibra Data Profiling. Managing data involves various processes, including collection, storage, and analysis. Collaborating with diverse teams typically leads to challenges, particularly in data-related tasks. Collibra mitigates these challenges by creating structured workflows that maximize productivity.
The automation of data profiling tasks allows teams to focus on higher-value activities. Routine tasks such as data cleansing and monitoring can be programmed within Collibra, streamlining operations. This automated approach reduces the risk of human error, which often plagues manual processes. As a result, not only do organizations save time, but they also enhance the quality of their data outputs.
The Role of Data Profiling in Data Governance
Data profiling plays a critical role in data governance by facilitating accurate and meaningful management of data assets. In today's data-driven environment, organizations face various challenges in maintaining data quality, compliance, and accessibility. Effective data profiling enhances the understanding of data characteristics, which is vital for any data governance initiative. Through systematic analysis, organizations can ensure their data is fit for intended use, thus supporting strategic goals and operational efficiency.
Integration with Data Governance Frameworks
Integrating data profiling into existing data governance frameworks is essential for achieving comprehensive oversight. When data profiling is performed, it uncovers patterns, standards, and anomalies present in the data. This information aids in defining data quality metrics and policies necessary for governance.
- Data Standardization: Profiling results inform data standardization processes, ensuring that data complies with internal and external standards.
- Policy Effectiveness: By reviewing profiling results, organizations can assess how efficiently existing governance policies function.
- Risk Management: Understanding data attributes helps identify potential risks related to data breaches and inaccuracies before they escalate into critical issues.
Organizations like Collibra offer tools to integrate profiling seamlessly into their governance structures. By embedding these practices, businesses enhance their accountability and transparency, reinforcing trust among stakeholders.
Compliance and Regulatory Considerations
Compliance with regulatory requirements is a paramount concern for organizations, especially in industries governed by strict data laws. Data profiling assists in this endeavor by ensuring organizations maintain a robust compliance posture.
- Regulatory Alignment: Profiling enables organizations to evaluate their data against regulatory frameworks such as GDPR and HIPAA, ensuring adherence to necessary guidelines.
- Audit Preparedness: Regular profiling creates an audit trail that serves as evidence of compliance efforts. Proper documentation can make audits less cumbersome.
- Mitigation of Legal Risks: By actively managing data quality through profiling, organizations reduce the risk of legal complications originating from data mismanagement or non-compliance.
"Data governance without profiling is like steering a ship without a compass. You may move, but you cannot ensure safety or sustainability."
Organizations engaging in regular data profiling not only fulfill compliance requirements but also build a culture of data stewardship. This proactive measure reflects an organization's commitment to data integrity and governance excellence. Ensuring alignment with regulations establishes a strong foundation upon which businesses can grow, innovate, and earn the trust of their clients and partners.
Collibra Data Profiling Workflow
The Collibra Data Profiling workflow serves as a critical framework that facilitates the comprehensive analysis of data sets within organizations. It systematically outlines the processes involved in identifying the quality, structure, and content of data, allowing users to derive actionable insights. Understanding this workflow is essential for maximizing the potential of data profiling, as it helps ensure that the data governance strategy is not only well-integrated but also effective.
This workflow is built on distinct yet interconnected stages, each of which plays a vital role in enhancing data governance practices. By following a structured approach, organizations can significantly improve data quality and facilitate informed decision-making. The focus of the workflow is not merely to assess data but to enhance its overall utility in various business contexts, thereby ensuring regulatory compliance and operational efficiency.
Step-by-Step Profiling Process
The step-by-step profiling process in Collibra involves several key stages:
- Data Source Identification: This first step entails pinpointing the data sources that require profiling. It could range from databases to cloud storage systems.
- Data Extraction and Ingestion: Once the sources are identified, data must be extracted and ingested into the Collibra platform for analysis. This process often necessitates the use of connectors or APIs.
- Profiling Configuration: In this phase, users configure the parameters of the profiling process. This includes selecting specific metrics and attributes relevant to the organization's goals.
- Running the Profiling Jobs: After configurations, it is time to execute the profiling jobs. During this stage, Collibra analyzes the datasets based on the predefined parameters.
- Results Gathering: Once profiling jobs are completed, the results are gathered for further analysis. This step is crucial for determining the quality and integrity of the data.
- Reporting: The final step involves creating reports that summarize the findings. These reports serve as a foundation for identifying areas that require attention and further action.
Adopting a systematic step-by-step process not only streamlines the data profiling efforts but also enhances reliability and repeatability in outcomes.
Analyzing Profiling Results
Analyzing the results generated from the Collibra profiling process is equally essential. This stage can uncover valuable insights about the data quality, compliance issues, and areas for improvement.
- Reviewing Quality Metrics: Users should first focus on quality metrics such as completeness, consistency, accuracy, and uniqueness. These metrics provide a quantifiable measure of how well the data serves its intended purpose.
- Identifying Data Anomalies: Profiling results often reveal anomalies such as duplicate entries or missing values. Recognizing such issues is the first step towards addressing them and improving data quality.
- Understanding Patterns: Analyzing patterns within the data can help reveal trends or correlations. This helps organizations in decision-making processes, guiding them to make evidence-based choices instead of relying on intuition.
- Taking Action: After assessing the results, it is crucial to take appropriate actions based on the findings. This could involve refining data collection methods, revising governance policies, or enhancing data entry processes to prevent future issues.
Important Note: Continuous monitoring of profiling results is recommended to maintain data quality over time.
Challenges and Limitations of Collibra Data Profiling
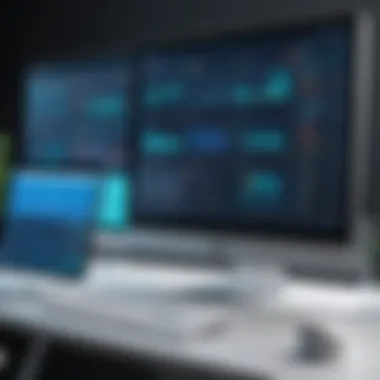
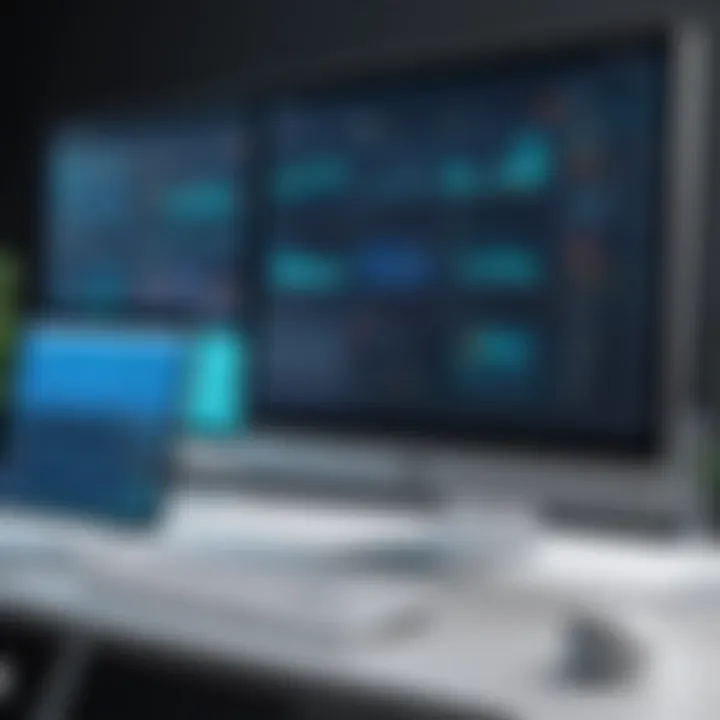
Understanding the challenges and limitations of Collibra Data Profiling facilitates a more complete understanding of the data management landscape. While the tool itself is powerful, it does not come without its issues. A clear awareness of these challenges enables professionals to prepare more effectively and optimize their use of data profiling techniques. Issues like data quality, system integration, and user training must be considered critically.
Common Issues in Data Profiling
There are several notable problems related to data profiling in Collibra. These problems can hinder not only the profiling process but also the overall success of data management initiatives. Here are some common issues:
- Data Quality Variability: Inconsistent data quality is critical. If data sources contain inaccuracies or discrepancies, profiling results may not be reliable. Data profiling may identify patterns, but flawed data affects decision-making.
- Integration Challenges: Collibra needs to connect with other systems and databases seamlessly. Often, integration requires substantial effort and fine-tuning. Compatibility issues can impede the flow of data, resulting in missed opportunities for insights.
- Scalability Limitations: As data volumes grow, Collibra data profiling can encounter performance bottlenecks. Large datasets might slow down profiling activities and delay data insight generation.
- User Adoption: The success of any tool is reliant on user proficiency. Collibra requires training for effective usage. If a team lacks knowledge or fails to adopt the tool properly, its benefits may not be fully realized.
- Dynamic Data Environments: In a rapidly changing data landscape, maintaining updated profiling continues to be a challenge. Rapid data introduction can complicate existing profiling efforts.
Mitigating Risks in Data Profiling
To address the challenges of data profiling within Collibra, organizations need to implement strategies to mitigate risks effectively. Here are key practices that can be beneficial:
- Establish Clear Data Standards: Define data quality standards prior to profiling. Clear guidelines help ensure consistent data input and reduce discrepancies.
- Conduct Regular Assessments: Regularly review data quality and profiling processes. Periodic assessments can identify potential gaps or weaknesses in data management efforts.
- Enable Training Programs: Offer comprehensive training to users. Effective training leads to better understanding of Collibra's capabilities and helps maximize its benefits.
- Invest in Integration Solutions: Utilize robust integration tools or platforms. Ensuring compatibility with data sources streamlines the profiling process.
- Monitor Performance Metrics: Actively track profiling metrics and performance. A stronger emphasis on analytics helps identify bottlenecks early.
"Embracing a proactive approach to data profiling can significantly elevate an organization's data governance maturity level."
Best Practices for Effective Data Profiling in Collibra
Effective data profiling in Collibra is vital for ensuring data quality and facilitating comprehensive understanding of datasets within an organization. In this section, we highlight the best practices that must be adopted to maximize the advantages of data profiling. Proper implementation of these practices leads to enhanced accuracy, reduced data risks, and ultimately, better decision-making.
Defining Clear Objectives
Before conducting data profiling, it is crucial to establish clear objectives. Defining objectives helps guide the profiling process, ensuring that all stakeholders understand the goals. This clarity aids in focusing efforts on specific metrics that matter most to the organization.
Some key points to consider include:
- Identifying the primary reasons for data profiling, such as compliance, quality improvement, or integration.
- Establishing success criteria that allow stakeholders to measure the outcomes of profiling activities.
- Communicating these objectives across teams to foster a unified approach.
Having well-defined objectives not only directs the process but also ensures that resources are allocated effectively. The outcomes become measurable, enabling organizations to evaluate the effectiveness of their data strategies.
Regular Updates and Maintenance
Regular updates and maintenance form an essential part of sustainable data profiling practices. Data profiles can change rapidly due to factors like new data influx, modifications in business rules, or external regulations. To keep data current and useful, it is necessary to maintain a schedule for updates.
Maintaining a consistent review schedule can include:
- Periodically reassessing the profiling metrics to align with enterprise goals.
- Updating profiles to reflect changes in data sources, structures, or quality expectations.
- Utilizing automated tools whenever possible to streamline this process.
Regular maintenance ensures that data profiling does not become outdated or irrelevant. Keeping profiles fresh allows organizations to stay agile and responsive in an ever-evolving data landscape.
"Data quality decreases over time; consistent maintenance is the only way to stay ahead." - Data Governance Expert
In summary, adhering to these best practices of defining clear objectives and ensuring regular updates helps in maximizing the potential of Collibra data profiling. This structured approach allows organizations to maintain high levels of data integrity, which is paramount in today's data-driven environments.
Case Studies of Organizations Using Collibra
Understanding how organizations utilize Collibra for data profiling offers invaluable insights into its practical applications. Case studies provide concrete examples of how different entities tackle data challenges and enhance operational efficiency. Through these studies, we can observe the diverse implementations of Collibra’s features in real-world scenarios. This understanding helps businesses appreciate the effectiveness of data profiling in fostering better data governance, quality, and decision-making.
Success Stories
Many organizations report significant successes after implementing Collibra for data profiling. For instance, a healthcare provider leveraged Collibra to enhance the quality of patient data. By conducting thorough data profiling, they identified inconsistencies in patient records that were previously overlooked. As a result, not only did patient safety improve, but the provider also ensured compliance with regulatory requirements, minimizing the risk of penalties.
Another notable success came from a financial services company. They utilized Collibra to establish a single source of truth for their data, enabling consistent reporting across departments. This success led to faster decision-making and increased trust in data among stakeholders.
The key benefits observed in these success stories include:
- Improved data accuracy: Organizations saw better alignment of their data elements, ensuring that stakeholders could rely on the information they accessed.
- Enhanced compliance: Regular data profiling helped maintain adherence to constantly evolving regulations, thereby reducing operational risks.
- Greater operational efficiency: Teams became more productive as they spent less time resolving data discrepancies and more time on analysis and strategy.
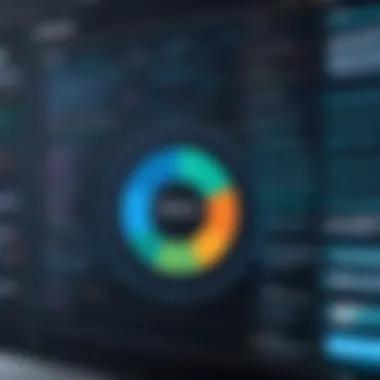
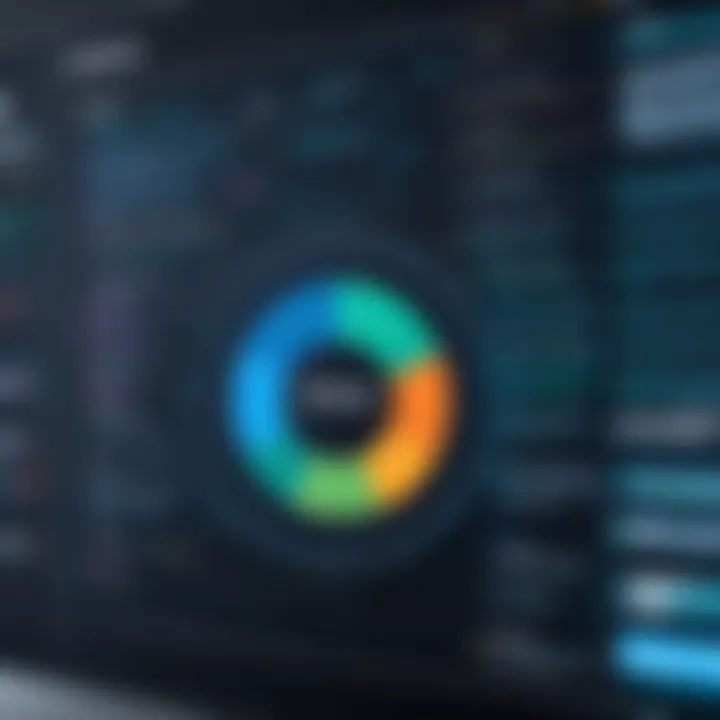
Lessons Learned
Examining the outcomes of Collibra implementations can reveal important lessons that organizations can apply when considering their own data profiling strategies. A common lesson is the necessity of having clear objectives when embarking on data profiling initiatives. Organizations that defined specific goals, such as improving data quality or achieving compliance, were more likely to experience positive outcomes.
Moreover, the importance of engaging all relevant stakeholders cannot be overstated. Organizations that involved key departments, such as compliance, operations, and IT during the initial stages of implementation, found that collaboration led to a richer understanding of data needs and better alignment with business objectives.
Key takeaways include:
- Prioritize cross-department collaboration: Involve various teams in defining data needs and potential challenges.
- Set measurable goals: Establish clear data profiling objectives to track progress and outcomes effectively.
- Utilize continuous feedback loops: Implement regular reviews of the profiling process, making iterative improvements based on stakeholder feedback.
"Understanding the practical application of Collibra data profiling through real-world examples highlights its value in organizations. These insights empower better decision-making and enhance operational efficiencies for future initiatives."
Organizations looking to implement data profiling can benefit immensely from learning from these experiences. Recognizing what worked and what did not aids in crafting a tailored approach relevant to their specific needs.
Future Trends in Data Profiling
Understanding the future trends in data profiling is crucial for organizations aiming to leverage their data assets effectively. As businesses recognize the importance of data-driven decision-making, the demand for robust data profiling tools is growing. The mechanisms and methodologies of data profiling evolve quickly, influenced by technological advancements and changing business needs. Staying informed on these trends can help organizations improve data quality and ensure compliance with regulations.
The Impact of Machine Learning
Machine learning is revolutionizing data profiling by introducing automated, advanced analytical techniques. Traditionally, data profiling relies on predefined rules and manual audits. However, with machine learning models, organizations can detect patterns and anomalies in vast datasets without extensive human intervention. This shift to automation not only increases efficiency but also reduces the likelihood of human error.
- Benefits of Machine Learning in Data Profiling:
- Enhanced Accuracy: Algorithms can adapt and learn from new data inputs, improving the precision of profiling processes.
- Real-time Insights: Organizations can gain insights more rapidly, enabling quicker response to data quality issues.
- Scalability: Machine learning empowers organizations to scale their data profiling efforts without proportional increases in resource allocation.
By integrating machine learning into Collibra's data profiling capabilities, users can streamline their profiling processes and enhance overall data governance. This technology will likely lead to more proactive data management, allowing organizations to address potential issues before they escalate.
Evolving Needs in Data Management
As the data landscape transforms, so too do the requirements for data profiling. Organizations are increasingly handling diverse datasets, including structured and unstructured data. The shift toward cloud computing and hybrid data environments presents both opportunities and challenges. Organizations must adapt their data profiling strategies to ensure they meet these evolving needs.
- Key Considerations for Data Management:
- Flexibility: Data profiling tools must be agile enough to accommodate varying data types and sources.
- Integration: Seamless integration with other data management tools remains essential for effective data governance.
- User Experience: An intuitive interface will become a priority, making it easier for non-technical users to engage with data profiling processes.
Maintaining relevance in this fast-paced environment will require continuous innovation in data profiling. As user demands shift, organizations utilizing Collibra must ensure they adapt their strategies and tools to stay ahead.
"Future trends in data profiling will not only shape how data is managed but also redefine the role of data within organizational frameworks."
Overall, the future of data profiling is complex yet exciting. The integration of machine learning and an adaptability to the changing landscape will enable organizations to harness the full potential of their data.
Epilogue
In wrapping up this exploration of Collibra Data Profiling, it is crucial to highlight the significance of this topic in the realm of data management and governance. The mechanisms of data profiling discussed throughout the article serve as foundational elements in ensuring data integrity and quality.
Effective data profiling transforms raw data into a valuable asset. It equips organizations with insights necessary to make informed decisions, thereby driving strategic initiatives. With Collibra's robust features and methodologies, businesses can enhance their data landscape significantly. Thus, the importance of investing in sound data profiling practices cannot be overstated. Not only does it align with compliance requirements, it also fosters a culture of data-driven decision making.
Summary of Key Points
The journey through Collibra Data Profiling has unveiled key aspects:
- Data Quality Enhancement: Profiles data to identify anomalies.
- Improved Decision-Making: Facilitates informed choices based on reliable data.
- Integration with Governance Frameworks: Supports regulatory compliance.
- Workflow Efficiency: Streamlines data management processes, reducing operational overhead.
These points illustrate how Collibra serves as a pivotal tool for organizations striving for data excellence.
Final Thoughts on Collibra Data Profiling
Collibra Data Profiling stands out as more than just a tool; it is a comprehensive framework designed for the modern data landscape. As organizations evolve and as data volumes grow, its importance will only increase. The role of effective profiling will be critical for not just achieving compliance, but for harnessing the full potential of data.
In essence, embracing Collibra Data Profiling offers organizations the opportunity to create a proactive data governance environment, which is essential for achieving long-term success. The future is data-centric, and tools like Collibra are at the forefront, enabling organizations to adapt and thrive in this changing landscape.
"Data quality is of utmost importance in today's digital age; without it, decision-making is compromised."
Investing time in mastering Collibra can open doors to significant business advantages.